Note
Go to the end to download the full example code. or to run this example in your browser via Binder
Searchlight analysis of face vs house recognition¶
Searchlight analysis requires fitting a classifier a large amount of times. As a result, it is an intrinsically slow method. In order to speed up computing, in this example, Searchlight is run only on one slice on the fMRI (see the generated figures).
Note
If you are using Nilearn with a version older than 0.9.0
,
then you should either upgrade your version or import maskers
from the input_data
module instead of the maskers
module.
That is, you should manually replace in the following example all occurrences of:
from nilearn.maskers import NiftiMasker
with:
from nilearn.input_data import NiftiMasker
Load Haxby dataset¶
import pandas as pd
from nilearn.datasets import fetch_haxby
from nilearn.image import get_data, load_img, new_img_like
# We fetch 2nd subject from haxby datasets (which is default)
haxby_dataset = fetch_haxby()
# print basic information on the dataset
print(f"Anatomical nifti image (3D) is located at: {haxby_dataset.mask}")
print(f"Functional nifti image (4D) is located at: {haxby_dataset.func[0]}")
fmri_filename = haxby_dataset.func[0]
labels = pd.read_csv(haxby_dataset.session_target[0], sep=" ")
y = labels["labels"]
run = labels["chunks"]
[fetch_haxby] Dataset found in /home/runner/nilearn_data/haxby2001
Anatomical nifti image (3D) is located at: /home/runner/nilearn_data/haxby2001/mask.nii.gz
Functional nifti image (4D) is located at: /home/runner/nilearn_data/haxby2001/subj2/bold.nii.gz
Restrict to faces and houses¶
from nilearn.image import index_img
condition_mask = y.isin(["face", "house"])
fmri_img = index_img(fmri_filename, condition_mask)
y, run = y[condition_mask], run[condition_mask]
Prepare masks¶
mask_img is the original mask
process_mask_img is a subset of mask_img, it contains the voxels that should be processed (we only keep the slice z = 29 and the back of the brain to speed up computation)
import numpy as np
mask_img = load_img(haxby_dataset.mask)
# .astype() makes a copy.
process_mask = get_data(mask_img).astype(int)
picked_slice = 29
process_mask[..., (picked_slice + 1) :] = 0
process_mask[..., :picked_slice] = 0
process_mask[:, 30:] = 0
process_mask_img = new_img_like(mask_img, process_mask)
/home/runner/work/nilearn/nilearn/examples/02_decoding/plot_haxby_searchlight.py:61: UserWarning: Data array used to create a new image contains 64-bit ints. This is likely due to creating the array with numpy and passing `int` as the `dtype`. Many tools such as FSL and SPM cannot deal with int64 in Nifti images, so for compatibility the data has been converted to int32.
process_mask_img = new_img_like(mask_img, process_mask)
Searchlight computation¶
# Make processing parallel
# /!\ As each thread will print its progress, n_jobs > 1 could mess up the
# information output.
n_jobs = 2
# Define the cross-validation scheme used for validation.
# Here we use a KFold cross-validation on the run, which corresponds to
# splitting the samples in 4 folds and make 4 runs using each fold as a test
# set once and the others as learning sets
from sklearn.model_selection import KFold
cv = KFold(n_splits=4)
import nilearn.decoding
# The radius is the one of the Searchlight sphere that will scan the volume
searchlight = nilearn.decoding.SearchLight(
mask_img,
process_mask_img=process_mask_img,
radius=5.6,
n_jobs=n_jobs,
verbose=1,
cv=cv,
)
searchlight.fit(fmri_img, y)
[Parallel(n_jobs=2)]: Using backend LokyBackend with 2 concurrent workers.
[Parallel(n_jobs=2)]: Done 2 out of 2 | elapsed: 13.7s remaining: 0.0s
[Parallel(n_jobs=2)]: Done 2 out of 2 | elapsed: 13.7s finished
Visualization¶
from nilearn import image
from nilearn.plotting import plot_img, plot_stat_map, show
# After fitting the searchlight, we can access the searchlight scores
# as a NIfTI image using the `scores_img_` attribute.
scores_img = searchlight.scores_img_
# Use the :term:`fMRI` mean image as a surrogate of anatomical data
mean_fmri = image.mean_img(fmri_img, copy_header=True)
# Because scores are not a zero-center test statistics,
# we cannot use plot_stat_map
plot_img(
scores_img,
bg_img=mean_fmri,
title="Searchlight scores image",
display_mode="z",
cut_coords=[-9],
vmin=0.2,
cmap="inferno",
threshold=0.2,
black_bg=True,
colorbar=True,
)
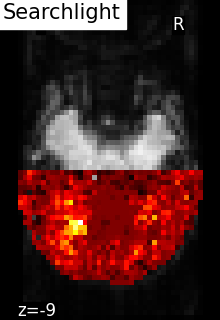
/home/runner/work/nilearn/nilearn/.tox/doc/lib/python3.9/site-packages/nilearn/plotting/displays/_slicers.py:1666: MatplotlibDeprecationWarning: Adding an axes using the same arguments as a previous axes currently reuses the earlier instance. In a future version, a new instance will always be created and returned. Meanwhile, this warning can be suppressed, and the future behavior ensured, by passing a unique label to each axes instance.
ax = fh.add_axes(
<nilearn.plotting.displays._slicers.ZSlicer object at 0x7f80f928bf10>
F-scores computation¶
from sklearn.feature_selection import f_classif
from nilearn.maskers import NiftiMasker
# For decoding, standardizing is often very important
nifti_masker = NiftiMasker(
mask_img=mask_img,
runs=run,
standardize="zscore_sample",
memory="nilearn_cache",
memory_level=1,
)
fmri_masked = nifti_masker.fit_transform(fmri_img)
_, p_values = f_classif(fmri_masked, y)
p_values = -np.log10(p_values)
p_values[p_values > 10] = 10
p_unmasked = get_data(nifti_masker.inverse_transform(p_values))
# F_score results
p_ma = np.ma.array(p_unmasked, mask=np.logical_not(process_mask))
f_score_img = new_img_like(mean_fmri, p_ma)
plot_stat_map(
f_score_img,
mean_fmri,
title="F-scores",
display_mode="z",
cut_coords=[-9],
cmap="inferno",
)
show()
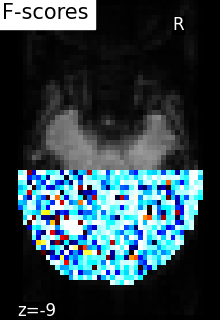
/home/runner/work/nilearn/nilearn/.tox/doc/lib/python3.9/site-packages/nilearn/plotting/displays/_slicers.py:1666: MatplotlibDeprecationWarning: Adding an axes using the same arguments as a previous axes currently reuses the earlier instance. In a future version, a new instance will always be created and returned. Meanwhile, this warning can be suppressed, and the future behavior ensured, by passing a unique label to each axes instance.
ax = fh.add_axes(
Total running time of the script: (0 minutes 25.033 seconds)
Estimated memory usage: 1010 MB