Note
Go to the end to download the full example code. or to run this example in your browser via Binder
Statistical testing of a second-level analysis¶
Perform a one-sample t-test on a bunch of images (a.k.a. second-level analysis in fMRI) and threshold the resulting statistical map.
This example is based on the so-called localizer dataset. It shows activation related to a mental computation task, as opposed to narrative sentence reading/listening.
Prepare some images for a simple t test¶
This is a simple manually performed second level analysis.
from nilearn import datasets
n_samples = 20
localizer_dataset = datasets.fetch_localizer_calculation_task(
n_subjects=n_samples, legacy_format=False
)
[get_dataset_dir] Dataset found in /home/runner/work/nilearn/nilearn/nilearn_data/brainomics_localizer
Get the set of individual statstical maps (contrast estimates)
Perform the second level analysis¶
First, we define a design matrix for the model. As the model is trivial (one-sample test), the design matrix is just one column with ones.
import pandas as pd
design_matrix = pd.DataFrame([1] * n_samples, columns=["intercept"])
Next, we specify and estimate the model.
from nilearn.glm.second_level import SecondLevelModel
second_level_model = SecondLevelModel(n_jobs=2).fit(
cmap_filenames, design_matrix=design_matrix
)
Compute the only possible contrast: the one-sample test. Since there is only one possible contrast, we don’t need to specify it in detail.
z_map = second_level_model.compute_contrast(output_type="z_score")
Threshold the resulting map without multiple comparisons correction, abs(z) > 3.29 (equivalent to p < 0.001), cluster size > 10 voxels.
from nilearn.image import threshold_img
threshold_img(
z_map,
threshold=3.29,
cluster_threshold=10,
two_sided=True,
copy_header=True,
)
<nibabel.nifti1.Nifti1Image object at 0x7ff03d6a6480>
This is equivalent to thresholding a z-statistic image with a false positive rate < .001, cluster size > 10 voxels.
from nilearn.glm import threshold_stats_img
thresholded_map1, threshold1 = threshold_stats_img(
z_map,
alpha=0.001,
height_control="fpr",
cluster_threshold=10,
two_sided=True,
)
Now use FDR <.05 (False Discovery Rate) and no cluster-level threshold.
thresholded_map2, threshold2 = threshold_stats_img(
z_map, alpha=0.05, height_control="fdr"
)
print(f"The FDR=.05 threshold is {threshold2:.3g}")
The FDR=.05 threshold is 2.37
Now use FWER <.05 (Family-Wise Error Rate) and no cluster-level threshold. As the data has not been intensively smoothed, we can use a simple Bonferroni correction.
thresholded_map3, threshold3 = threshold_stats_img(
z_map, alpha=0.05, height_control="bonferroni"
)
print(f"The p<.05 Bonferroni-corrected threshold is {threshold3:.3g}")
The p<.05 Bonferroni-corrected threshold is 4.88
Visualize the results¶
First, the unthresholded map.
from nilearn import plotting
display = plotting.plot_stat_map(z_map, title="Raw z map")
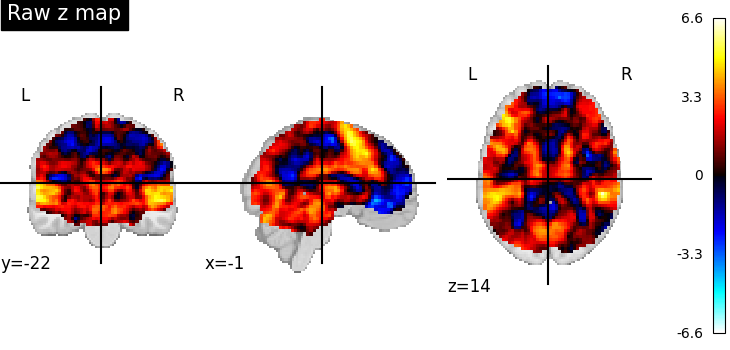
Second, the p<.001 uncorrected-thresholded map (with only clusters > 10 voxels).
plotting.plot_stat_map(
thresholded_map1,
cut_coords=display.cut_coords,
threshold=threshold1,
title="Thresholded z map, fpr <.001, clusters > 10 voxels",
)
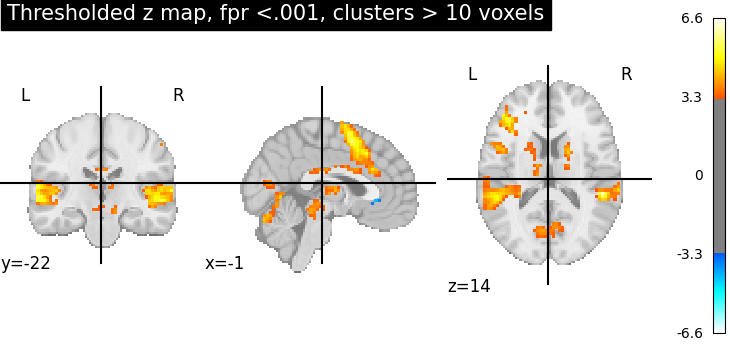
<nilearn.plotting.displays._slicers.OrthoSlicer object at 0x7ff05afef440>
Third, the fdr-thresholded map.
plotting.plot_stat_map(
thresholded_map2,
cut_coords=display.cut_coords,
title="Thresholded z map, expected fdr = .05",
threshold=threshold2,
)
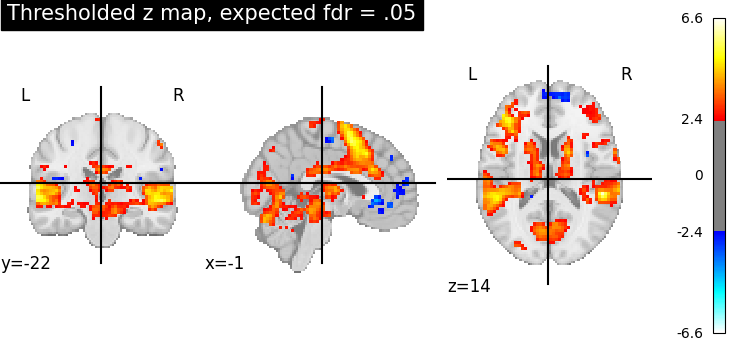
<nilearn.plotting.displays._slicers.OrthoSlicer object at 0x7ff05afeebd0>
Fourth, the Bonferroni-thresholded map.
plotting.plot_stat_map(
thresholded_map3,
cut_coords=display.cut_coords,
title="Thresholded z map, expected fwer < .05",
threshold=threshold3,
)
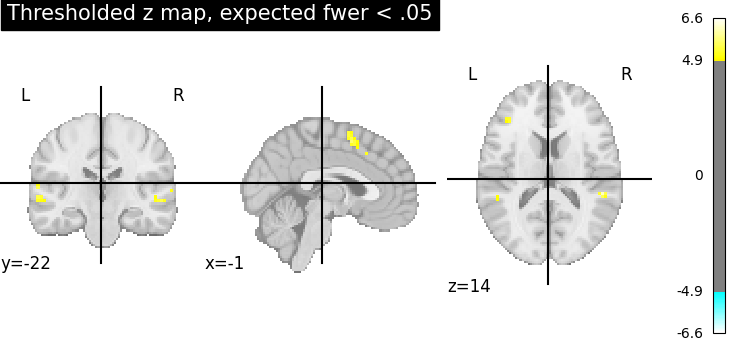
<nilearn.plotting.displays._slicers.OrthoSlicer object at 0x7ff0408b3980>
These different thresholds correspond to different statistical guarantees: in the FWER-corrected image there is only a probability smaller than .05 of observing any false positive voxel. In the FDR-corrected image, 5% of the voxels found are likely to be false positive. In the uncorrected image, one expects a few tens of false positive voxels.
Total running time of the script: (0 minutes 10.511 seconds)
Estimated memory usage: 169 MB