Note
Go to the end to download the full example code. or to run this example in your browser via Binder
Functional connectivity predicts age group¶
This example compares different kinds of functional connectivity between regions of interest : correlation, partial correlation, and tangent space embedding.
The resulting connectivity coefficients can be used to discriminate children from adults. In general, the tangent space embedding outperforms the standard correlations: see Dadi et al.[1] for a careful study.
Note
If you are using Nilearn with a version older than 0.9.0
,
then you should either upgrade your version or import maskers
from the input_data
module instead of the maskers
module.
That is, you should manually replace in the following example all occurrences of:
from nilearn.maskers import NiftiMasker
with:
from nilearn.input_data import NiftiMasker
try:
import matplotlib.pyplot as plt
except ImportError:
raise RuntimeError("This script needs the matplotlib library")
Load brain development fMRI dataset and MSDL atlas¶
We study only 60 subjects from the dataset, to save computation time.
from nilearn import datasets
development_dataset = datasets.fetch_development_fmri(n_subjects=60)
[fetch_development_fmri] Dataset found in
/home/runner/nilearn_data/development_fmri
[fetch_development_fmri] Dataset found in
/home/runner/nilearn_data/development_fmri/development_fmri
[fetch_development_fmri] Dataset found in
/home/runner/nilearn_data/development_fmri/development_fmri
[fetch_development_fmri] Downloading data from
https://osf.io/download/5c8ff3f72286e80019c3c1af/ ...
[fetch_development_fmri] ...done. (2 seconds, 0 min)
[fetch_development_fmri] Downloading data from
https://osf.io/download/5c8ff3f92286e80018c3e463/ ...
[fetch_development_fmri] ...done. (1 seconds, 0 min)
[fetch_development_fmri] Downloading data from
https://osf.io/download/5c8ff3f6a743a90017608171/ ...
[fetch_development_fmri] ...done. (2 seconds, 0 min)
[fetch_development_fmri] Downloading data from
https://osf.io/download/5c8ff3f64712b400183b70d8/ ...
[fetch_development_fmri] ...done. (1 seconds, 0 min)
[fetch_development_fmri] Downloading data from
https://osf.io/download/5c8ff3eb2286e80019c3c198/ ...
[fetch_development_fmri] ...done. (2 seconds, 0 min)
[fetch_development_fmri] Downloading data from
https://osf.io/download/5c8ff3ed2286e80017c41b56/ ...
[fetch_development_fmri] ...done. (2 seconds, 0 min)
[fetch_development_fmri] Downloading data from
https://osf.io/download/5c8ff3ee2286e80016c3c379/ ...
[fetch_development_fmri] ...done. (1 seconds, 0 min)
[fetch_development_fmri] Downloading data from
https://osf.io/download/5c8ff3ee4712b400183b70c3/ ...
[fetch_development_fmri] ...done. (1 seconds, 0 min)
[fetch_development_fmri] Downloading data from
https://osf.io/download/5c8ff3efa743a9001660a0d5/ ...
[fetch_development_fmri] ...done. (2 seconds, 0 min)
[fetch_development_fmri] Downloading data from
https://osf.io/download/5c8ff3f14712b4001a3b560e/ ...
[fetch_development_fmri] ...done. (1 seconds, 0 min)
[fetch_development_fmri] Downloading data from
https://osf.io/download/5c8ff3f1a743a90017608164/ ...
[fetch_development_fmri] ...done. (1 seconds, 0 min)
[fetch_development_fmri] Downloading data from
https://osf.io/download/5c8ff3f12286e80016c3c37e/ ...
[fetch_development_fmri] ...done. (2 seconds, 0 min)
[fetch_development_fmri] Downloading data from
https://osf.io/download/5c8ff3f34712b4001a3b5612/ ...
[fetch_development_fmri] ...done. (1 seconds, 0 min)
[fetch_development_fmri] Downloading data from
https://osf.io/download/5c8ff3f7a743a90019606cdf/ ...
[fetch_development_fmri] ...done. (1 seconds, 0 min)
[fetch_development_fmri] Downloading data from
https://osf.io/download/5cb470153992690018133d3b/ ...
[fetch_development_fmri] ...done. (1 seconds, 0 min)
[fetch_development_fmri] Downloading data from
https://osf.io/download/5cb46e793992690017108eb9/ ...
[fetch_development_fmri] ...done. (1 seconds, 0 min)
[fetch_development_fmri] Downloading data from
https://osf.io/download/5cb47038353c5800199ac9a2/ ...
[fetch_development_fmri] ...done. (1 seconds, 0 min)
[fetch_development_fmri] Downloading data from
https://osf.io/download/5cb46e85a3bc97001aeff750/ ...
[fetch_development_fmri] ...done. (2 seconds, 0 min)
[fetch_development_fmri] Downloading data from
https://osf.io/download/5cb4701c3992690018133d49/ ...
[fetch_development_fmri] ...done. (1 seconds, 0 min)
[fetch_development_fmri] Downloading data from
https://osf.io/download/5cb46e1c3992690018133a9e/ ...
[fetch_development_fmri] ...done. (1 seconds, 0 min)
[fetch_development_fmri] Downloading data from
https://osf.io/download/5c8ff39aa743a900176080bf/ ...
[fetch_development_fmri] ...done. (1 seconds, 0 min)
[fetch_development_fmri] Downloading data from
https://osf.io/download/5c8ff39d4712b400193b5b89/ ...
[fetch_development_fmri] ...done. (1 seconds, 0 min)
[fetch_development_fmri] Downloading data from
https://osf.io/download/5cb4703039926900160f6b3e/ ...
[fetch_development_fmri] ...done. (1 seconds, 0 min)
[fetch_development_fmri] Downloading data from
https://osf.io/download/5cb46e4d353c58001b9cb325/ ...
[fetch_development_fmri] ...done. (2 seconds, 0 min)
[fetch_development_fmri] Downloading data from
https://osf.io/download/5cb4700af2be3c0017056f69/ ...
[fetch_development_fmri] ...done. (1 seconds, 0 min)
[fetch_development_fmri] Downloading data from
https://osf.io/download/5cb46e0cf2be3c001801f757/ ...
[fetch_development_fmri] ...done. (1 seconds, 0 min)
[fetch_development_fmri] Downloading data from
https://osf.io/download/5cb4702b39926900171090e4/ ...
[fetch_development_fmri] ...done. (1 seconds, 0 min)
[fetch_development_fmri] Downloading data from
https://osf.io/download/5cb46e35f2be3c00190305ff/ ...
[fetch_development_fmri] ...done. (1 seconds, 0 min)
[fetch_development_fmri] Downloading data from
https://osf.io/download/5c8ff3a12286e80017c41a48/ ...
[fetch_development_fmri] ...done. (1 seconds, 0 min)
[fetch_development_fmri] Downloading data from
https://osf.io/download/5c8ff3a12286e80016c3c2fc/ ...
[fetch_development_fmri] ...done. (1 seconds, 0 min)
[fetch_development_fmri] Downloading data from
https://osf.io/download/5cb4701ff2be3c0017056fad/ ...
[fetch_development_fmri] ...done. (1 seconds, 0 min)
[fetch_development_fmri] Downloading data from
https://osf.io/download/5cb46e0339926900160f6930/ ...
[fetch_development_fmri] ...done. (1 seconds, 0 min)
[fetch_development_fmri] Downloading data from
https://osf.io/download/5c8ff39fa743a90018606e2f/ ...
[fetch_development_fmri] ...done. (1 seconds, 0 min)
[fetch_development_fmri] Downloading data from
https://osf.io/download/5c8ff3a34712b4001a3b55a3/ ...
[fetch_development_fmri] ...done. (1 seconds, 0 min)
[fetch_development_fmri] Downloading data from
https://osf.io/download/5cb4703439926900160f6b43/ ...
[fetch_development_fmri] ...done. (1 seconds, 0 min)
[fetch_development_fmri] Downloading data from
https://osf.io/download/5cb46e40f2be3c001801f77f/ ...
[fetch_development_fmri] ...done. (1 seconds, 0 min)
[fetch_development_fmri] Downloading data from
https://osf.io/download/5c8ff3a34712b400193b5b92/ ...
[fetch_development_fmri] ...done. (1 seconds, 0 min)
[fetch_development_fmri] Downloading data from
https://osf.io/download/5c8ff3a84712b400183b7048/ ...
[fetch_development_fmri] ...done. (1 seconds, 0 min)
[fetch_development_fmri] Downloading data from
https://osf.io/download/5c8ff39aa743a90018606e21/ ...
[fetch_development_fmri] ...done. (1 seconds, 0 min)
[fetch_development_fmri] Downloading data from
https://osf.io/download/5c8ff39aa743a900176080ba/ ...
[fetch_development_fmri] ...done. (1 seconds, 0 min)
[fetch_development_fmri] Downloading data from
https://osf.io/download/5c8ff3a72286e80017c41a54/ ...
[fetch_development_fmri] ...done. (1 seconds, 0 min)
[fetch_development_fmri] Downloading data from
https://osf.io/download/5c8ff3a7a743a90018606e42/ ...
[fetch_development_fmri] ...done. (2 seconds, 0 min)
[fetch_development_fmri] Downloading data from
https://osf.io/download/5cb4702639926900190faf1d/ ...
[fetch_development_fmri] ...done. (1 seconds, 0 min)
[fetch_development_fmri] Downloading data from
https://osf.io/download/5cb46e3f353c5800199ac787/ ...
[fetch_development_fmri] ...done. (1 seconds, 0 min)
[fetch_development_fmri] Downloading data from
https://osf.io/download/5c8ff39ca743a90019606c50/ ...
[fetch_development_fmri] ...done. (1 seconds, 0 min)
[fetch_development_fmri] Downloading data from
https://osf.io/download/5c8ff3a2a743a9001660a048/ ...
[fetch_development_fmri] ...done. (1 seconds, 0 min)
[fetch_development_fmri] Downloading data from
https://osf.io/download/5cb47020f2be3c0019030968/ ...
[fetch_development_fmri] ...done. (1 seconds, 0 min)
[fetch_development_fmri] Downloading data from
https://osf.io/download/5cb46e6f353c58001a9b3311/ ...
[fetch_development_fmri] ...done. (1 seconds, 0 min)
[fetch_development_fmri] Downloading data from
https://osf.io/download/5cb47016a3bc970018f1fc88/ ...
[fetch_development_fmri] ...done. (2 seconds, 0 min)
[fetch_development_fmri] Downloading data from
https://osf.io/download/5cb46e6ba3bc970019f07152/ ...
[fetch_development_fmri] ...done. (1 seconds, 0 min)
[fetch_development_fmri] Downloading data from
https://osf.io/download/5c8ff395a743a900176080af/ ...
[fetch_development_fmri] ...done. (2 seconds, 0 min)
[fetch_development_fmri] Downloading data from
https://osf.io/download/5c8ff3964712b400193b5b7d/ ...
[fetch_development_fmri] ...done. (2 seconds, 0 min)
[fetch_development_fmri] Downloading data from
https://osf.io/download/5cb47057f2be3c0019030a1f/ ...
[fetch_development_fmri] ...done. (2 seconds, 0 min)
[fetch_development_fmri] Downloading data from
https://osf.io/download/5cb46e63f2be3c0017056ba9/ ...
[fetch_development_fmri] ...done. (1 seconds, 0 min)
[fetch_development_fmri] Downloading data from
https://osf.io/download/5cb4704af2be3c001705703b/ ...
[fetch_development_fmri] ...done. (1 seconds, 0 min)
[fetch_development_fmri] Downloading data from
https://osf.io/download/5cb46e7a353c58001a9b3324/ ...
[fetch_development_fmri] ...done. (1 seconds, 0 min)
[fetch_development_fmri] Downloading data from
https://osf.io/download/5c8ff3952286e80016c3c2e7/ ...
[fetch_development_fmri] ...done. (1 seconds, 0 min)
[fetch_development_fmri] Downloading data from
https://osf.io/download/5c8ff3954712b400193b5b79/ ...
[fetch_development_fmri] ...done. (2 seconds, 0 min)
[fetch_development_fmri] Downloading data from
https://osf.io/download/5c8ff399a743a9001660a031/ ...
[fetch_development_fmri] ...done. (1 seconds, 0 min)
[fetch_development_fmri] Downloading data from
https://osf.io/download/5c8ff3982286e80017c41a29/ ...
[fetch_development_fmri] ...done. (1 seconds, 0 min)
We use probabilistic regions of interest (ROIs) from the MSDL atlas.
from nilearn.maskers import NiftiMapsMasker
msdl_data = datasets.fetch_atlas_msdl()
msdl_coords = msdl_data.region_coords
masker = NiftiMapsMasker(
msdl_data.maps,
resampling_target="data",
t_r=2,
detrend=True,
low_pass=0.1,
high_pass=0.01,
memory="nilearn_cache",
memory_level=1,
standardize="zscore_sample",
standardize_confounds=True,
).fit()
masked_data = [
masker.transform(func, confounds)
for (func, confounds) in zip(
development_dataset.func, development_dataset.confounds
)
]
[fetch_atlas_msdl] Dataset found in /home/runner/nilearn_data/msdl_atlas
What kind of connectivity is most powerful for classification?¶
we will use connectivity matrices as features to distinguish children from adults. We use cross-validation and measure classification accuracy to compare the different kinds of connectivity matrices.
# prepare the classification pipeline
from sklearn.dummy import DummyClassifier
from sklearn.model_selection import GridSearchCV
from sklearn.pipeline import Pipeline
from sklearn.svm import LinearSVC
from nilearn.connectome import ConnectivityMeasure
kinds = ["correlation", "partial correlation", "tangent"]
pipe = Pipeline(
[
(
"connectivity",
ConnectivityMeasure(
vectorize=True,
standardize="zscore_sample",
),
),
(
"classifier",
GridSearchCV(LinearSVC(dual=True), {"C": [0.1, 1.0, 10.0]}, cv=5),
),
]
)
param_grid = [
{"classifier": [DummyClassifier(strategy="most_frequent")]},
{"connectivity__kind": kinds},
]
We use random splits of the subjects into training/testing sets. StratifiedShuffleSplit allows preserving the proportion of children in the test set.
from sklearn.model_selection import StratifiedShuffleSplit
from sklearn.preprocessing import LabelEncoder
groups = development_dataset.phenotypic["Child_Adult"].to_list()
classes = LabelEncoder().fit_transform(groups)
cv = StratifiedShuffleSplit(n_splits=30, random_state=0, test_size=10)
gs = GridSearchCV(
pipe,
param_grid,
scoring="accuracy",
cv=cv,
verbose=1,
refit=False,
n_jobs=2,
)
gs.fit(masked_data, classes)
mean_scores = gs.cv_results_["mean_test_score"]
scores_std = gs.cv_results_["std_test_score"]
Fitting 30 folds for each of 4 candidates, totalling 120 fits
display the results
plt.figure(figsize=(6, 4), constrained_layout=True)
positions = [0.1, 0.2, 0.3, 0.4]
plt.barh(positions, mean_scores, align="center", height=0.05, xerr=scores_std)
yticks = ["dummy", *list(gs.cv_results_["param_connectivity__kind"].data[1:])]
yticks = [t.replace(" ", "\n") for t in yticks]
plt.yticks(positions, yticks)
plt.xlabel("Classification accuracy")
plt.gca().grid(True)
plt.gca().set_axisbelow(True)
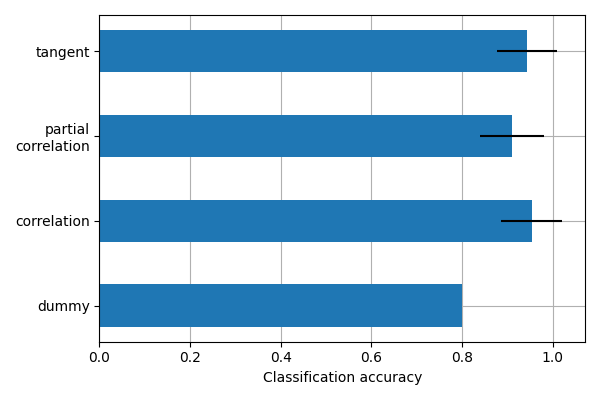
This is a small example to showcase nilearn features. In practice such comparisons need to be performed on much larger cohorts and several datasets. Dadi et al.[1] showed that across many cohorts and clinical questions, the tangent kind should be preferred.
plt.show()
References¶
Total running time of the script: (2 minutes 14.903 seconds)
Estimated memory usage: 1242 MB