Note
This page is a reference documentation. It only explains the class signature, and not how to use it. Please refer to the user guide for the big picture.
nilearn.maskers.BaseMasker¶
- class nilearn.maskers.BaseMasker[source]¶
Base class for NiftiMaskers.
- __init__(*args, **kwargs)¶
- abstract transform_single_imgs(imgs, confounds=None, sample_mask=None, copy=True)[source]¶
Extract signals from a single 4D niimg.
- Parameters:
- imgs3D/4D Niimg-like object
See Input and output: neuroimaging data representation. Images to process. If a 3D niimg is provided, a singleton dimension will be added to the output to represent the single scan in the niimg.
- confoundsCSV file or array-like, default=None
This parameter is passed to signal.clean. Please see the related documentation for details. shape: (number of scans, number of confounds)
- sample_maskAny type compatible with numpy-array indexing, default=None
shape: (number of scans - number of volumes removed, ) Masks the niimgs along time/fourth dimension to perform scrubbing (remove volumes with high motion) and/or non-steady-state volumes. This parameter is passed to signal.clean.
Added in version 0.8.0.
- copy
bool
, default=True Indicates whether a copy is returned or not.
- Returns:
- region_signals2D numpy.ndarray
Signal for each element. shape: (number of scans, number of elements)
- Warns:
- DeprecationWarning
If a 3D niimg input is provided, the current behavior (adding a singleton dimension to produce a 2D array) is deprecated. Starting in version 0.12, a 1D array will be returned for 3D inputs.
- transform(imgs, confounds=None, sample_mask=None)[source]¶
Apply mask, spatial and temporal preprocessing.
- Parameters:
- imgs3D/4D Niimg-like object
See Input and output: neuroimaging data representation. Images to process. If a 3D niimg is provided, a singleton dimension will be added to the output to represent the single scan in the niimg.
- confoundsCSV file or array-like, default=None
This parameter is passed to signal.clean. Please see the related documentation for details. shape: (number of scans, number of confounds)
- sample_maskAny type compatible with numpy-array indexing, default=None
shape: (number of scans - number of volumes removed, ) Masks the niimgs along time/fourth dimension to perform scrubbing (remove volumes with high motion) and/or non-steady-state volumes. This parameter is passed to signal.clean.
Added in version 0.8.0.
- Returns:
- region_signals2D numpy.ndarray
Signal for each element. shape: (number of scans, number of elements)
- Warns:
- DeprecationWarning
If a 3D niimg input is provided, the current behavior (adding a singleton dimension to produce a 2D array) is deprecated. Starting in version 0.12, a 1D array will be returned for 3D inputs.
- fit_transform(X, y=None, confounds=None, sample_mask=None, **fit_params)[source]¶
Fit to data, then transform it.
- Parameters:
- XNiimg-like object
- ynumpy array of shape [n_samples], default=None
Target values.
- confounds
list
of confounds, default=None List of confounds (2D arrays or filenames pointing to CSV files). Must be of same length than imgs_list.
- sample_mask
list
of sample_mask, default=None List of sample_mask (1D arrays) if scrubbing motion outliers. Must be of same length than imgs_list.
Added in version 0.8.0.
- Returns:
- X_newnumpy array of shape [n_samples, n_features_new]
Transformed array.
- inverse_transform(X)[source]¶
Transform the 2D data matrix back to an image in brain space.
This step only performs spatial unmasking, without inverting any additional processing performed by
transform
, such as temporal filtering or smoothing.- Parameters:
- X1D/2D
numpy.ndarray
Signal for each element in the mask. If a 1D array is provided, then the shape should be (number of elements,), and a 3D img will be returned. If a 2D array is provided, then the shape should be (number of scans, number of elements), and a 4D img will be returned. See Input and output: neuroimaging data representation.
- X1D/2D
- Returns:
- imgTransformed image in brain space.
- get_metadata_routing()¶
Get metadata routing of this object.
Please check User Guide on how the routing mechanism works.
- Returns:
- routingMetadataRequest
A
MetadataRequest
encapsulating routing information.
- get_params(deep=True)¶
Get parameters for this estimator.
- Parameters:
- deepbool, default=True
If True, will return the parameters for this estimator and contained subobjects that are estimators.
- Returns:
- paramsdict
Parameter names mapped to their values.
- set_fit_request(*, imgs='$UNCHANGED$')¶
Request metadata passed to the
fit
method.Note that this method is only relevant if
enable_metadata_routing=True
(seesklearn.set_config
). Please see User Guide on how the routing mechanism works.The options for each parameter are:
True
: metadata is requested, and passed tofit
if provided. The request is ignored if metadata is not provided.False
: metadata is not requested and the meta-estimator will not pass it tofit
.None
: metadata is not requested, and the meta-estimator will raise an error if the user provides it.str
: metadata should be passed to the meta-estimator with this given alias instead of the original name.
The default (
sklearn.utils.metadata_routing.UNCHANGED
) retains the existing request. This allows you to change the request for some parameters and not others.Added in version 1.3.
Note
This method is only relevant if this estimator is used as a sub-estimator of a meta-estimator, e.g. used inside a
Pipeline
. Otherwise it has no effect.- Parameters:
- imgsstr, True, False, or None, default=sklearn.utils.metadata_routing.UNCHANGED
Metadata routing for
imgs
parameter infit
.
- Returns:
- selfobject
The updated object.
- set_output(*, transform=None)¶
Set output container.
See Introducing the set_output API for an example on how to use the API.
- Parameters:
- transform{“default”, “pandas”}, default=None
Configure output of transform and fit_transform.
“default”: Default output format of a transformer
“pandas”: DataFrame output
“polars”: Polars output
None: Transform configuration is unchanged
Added in version 1.4: “polars” option was added.
- Returns:
- selfestimator instance
Estimator instance.
- set_params(**params)¶
Set the parameters of this estimator.
The method works on simple estimators as well as on nested objects (such as
Pipeline
). The latter have parameters of the form<component>__<parameter>
so that it’s possible to update each component of a nested object.- Parameters:
- **paramsdict
Estimator parameters.
- Returns:
- selfestimator instance
Estimator instance.
- set_transform_request(*, confounds='$UNCHANGED$', imgs='$UNCHANGED$', sample_mask='$UNCHANGED$')¶
Request metadata passed to the
transform
method.Note that this method is only relevant if
enable_metadata_routing=True
(seesklearn.set_config
). Please see User Guide on how the routing mechanism works.The options for each parameter are:
True
: metadata is requested, and passed totransform
if provided. The request is ignored if metadata is not provided.False
: metadata is not requested and the meta-estimator will not pass it totransform
.None
: metadata is not requested, and the meta-estimator will raise an error if the user provides it.str
: metadata should be passed to the meta-estimator with this given alias instead of the original name.
The default (
sklearn.utils.metadata_routing.UNCHANGED
) retains the existing request. This allows you to change the request for some parameters and not others.Added in version 1.3.
Note
This method is only relevant if this estimator is used as a sub-estimator of a meta-estimator, e.g. used inside a
Pipeline
. Otherwise it has no effect.- Parameters:
- confoundsstr, True, False, or None, default=sklearn.utils.metadata_routing.UNCHANGED
Metadata routing for
confounds
parameter intransform
.- imgsstr, True, False, or None, default=sklearn.utils.metadata_routing.UNCHANGED
Metadata routing for
imgs
parameter intransform
.- sample_maskstr, True, False, or None, default=sklearn.utils.metadata_routing.UNCHANGED
Metadata routing for
sample_mask
parameter intransform
.
- Returns:
- selfobject
The updated object.
Examples using nilearn.maskers.BaseMasker
¶
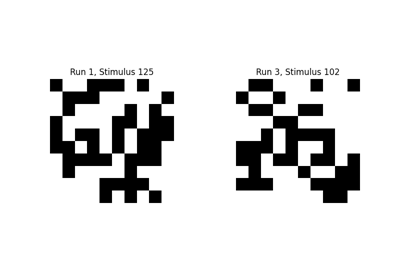
Encoding models for visual stimuli from Miyawaki et al. 2008
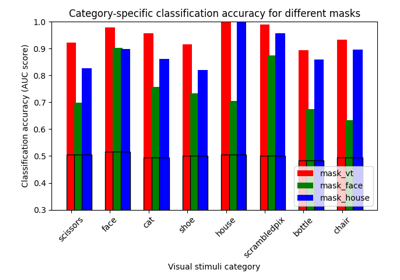
ROI-based decoding analysis in Haxby et al. dataset
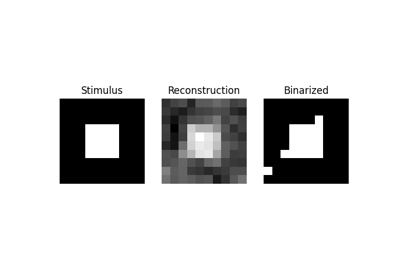
Reconstruction of visual stimuli from Miyawaki et al. 2008
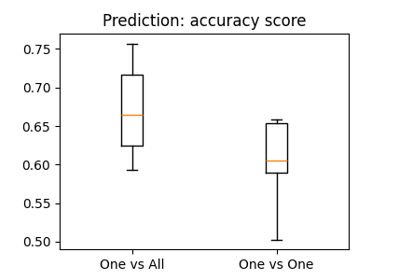
The haxby dataset: different multi-class strategies
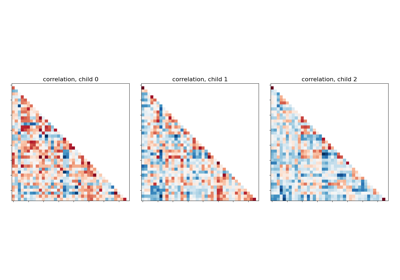
Classification of age groups using functional connectivity
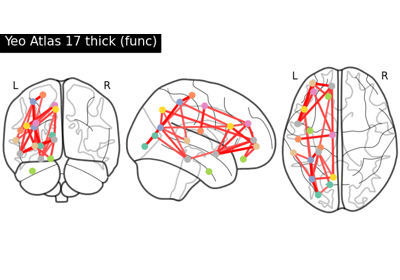
Comparing connectomes on different reference atlases
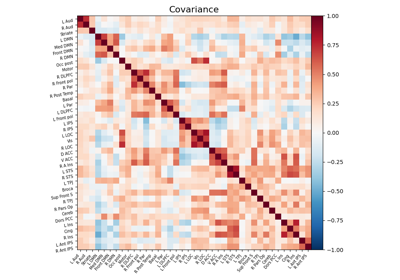
Computing a connectome with sparse inverse covariance
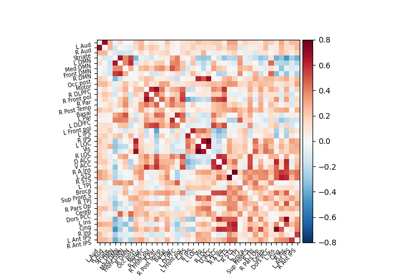
Extracting signals of a probabilistic atlas of functional regions
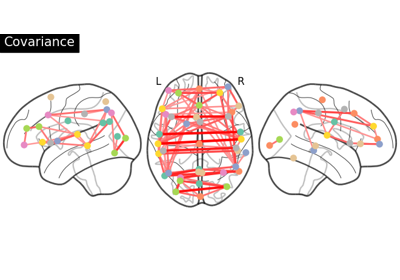
Group Sparse inverse covariance for multi-subject connectome
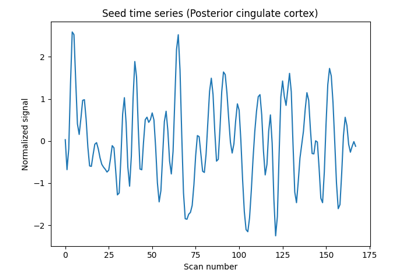
Producing single subject maps of seed-to-voxel correlation
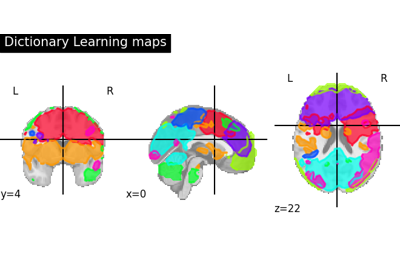
Regions extraction using dictionary learning and functional connectomes
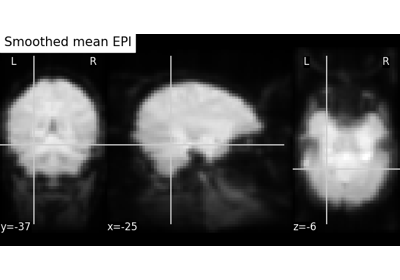
Computing a Region of Interest (ROI) mask manually
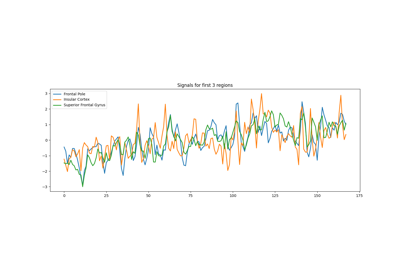
Extracting signals from brain regions using the NiftiLabelsMasker
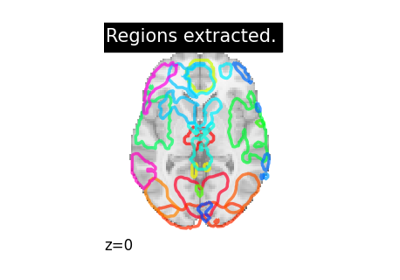
Regions Extraction of Default Mode Networks using Smith Atlas
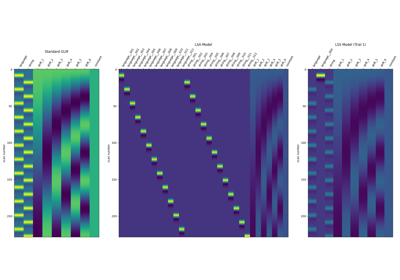
Beta-Series Modeling for Task-Based Functional Connectivity and Decoding
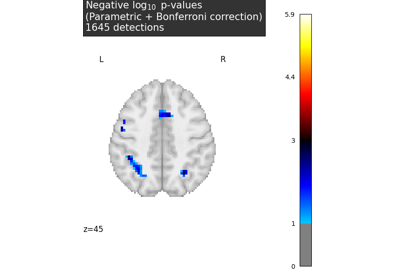
Massively univariate analysis of a calculation task from the Localizer dataset
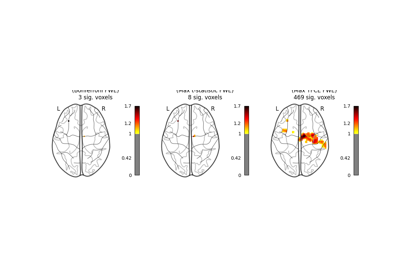
Massively univariate analysis of a motor task from the Localizer dataset
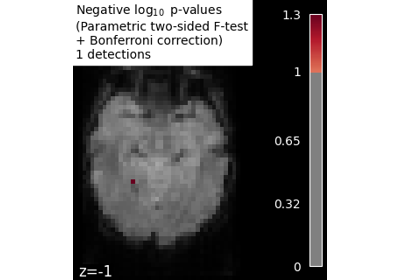
Massively univariate analysis of face vs house recognition
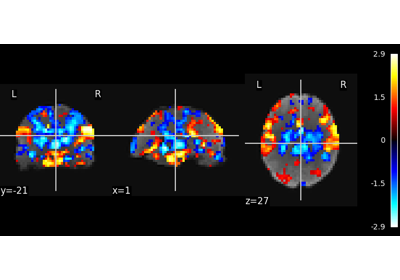
Multivariate decompositions: Independent component analysis of fMRI