Note
Go to the end to download the full example code. or to run this example in your browser via Binder
Default Mode Network extraction of ADHD dataset¶
This example shows a full step-by-step workflow of fitting a GLM to signal extracted from a seed on the Posterior Cingulate Cortex and saving the results. More precisely, this example shows how to use a signal extracted from a seed region as the regressor in a GLM to determine the correlation of each region in the dataset with the seed region.
More specifically:
A sequence of fMRI volumes are loaded.
A design matrix with the Posterior Cingulate Cortex seed is defined.
A GLM is applied to the dataset (effect/covariance, then contrast estimation).
The Default Mode Network is displayed.
Note
If you are using Nilearn with a version older than 0.9.0
, then you should either upgrade your version or import maskers from the input_data
module instead of the maskers
module.
That is, you should manually replace in the following example all occurrences of:
from nilearn.maskers import NiftiMasker
with:
from nilearn.input_data import NiftiMasker
import numpy as np
from nilearn import datasets, plotting
from nilearn.glm.first_level import (
FirstLevelModel,
make_first_level_design_matrix,
)
from nilearn.maskers import NiftiSpheresMasker
Prepare data and analysis parameters¶
Prepare the data.
adhd_dataset = datasets.fetch_adhd(n_subjects=1)
# Prepare timing
t_r = 2.0
slice_time_ref = 0.0
n_scans = 176
# Prepare seed
pcc_coords = (0, -53, 26)
[get_dataset_dir] Dataset found in /home/remi/nilearn_data/adhd
Extract the seed region’s time course¶
Extract the time course of the seed region.
seed_masker = NiftiSpheresMasker(
[pcc_coords],
radius=10,
detrend=True,
standardize="zscore_sample",
low_pass=0.1,
high_pass=0.01,
t_r=2.0,
memory="nilearn_cache",
memory_level=1,
verbose=0,
)
seed_time_series = seed_masker.fit_transform(adhd_dataset.func[0])
frametimes = np.linspace(0, (n_scans - 1) * t_r, n_scans)
Plot the time course of the seed region.
import matplotlib.pyplot as plt
fig = plt.figure(figsize=(9, 3))
ax = fig.add_subplot(111)
ax.plot(frametimes, seed_time_series, linewidth=2, label="seed region")
ax.legend(loc=2)
ax.set_title("Time course of the seed region")
plt.show()
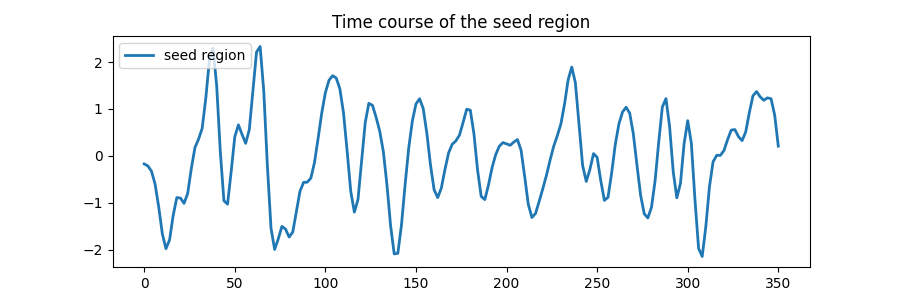
Estimate contrasts¶
Specify the contrasts.
design_matrix = make_first_level_design_matrix(
frametimes,
hrf_model="spm",
add_regs=seed_time_series,
add_reg_names=["pcc_seed"],
)
dmn_contrast = np.array([1] + [0] * (design_matrix.shape[1] - 1))
contrasts = {"seed_based_glm": dmn_contrast}
Perform first level analysis¶
Setup and fit GLM.
first_level_model = FirstLevelModel(t_r=t_r, slice_time_ref=slice_time_ref)
first_level_model = first_level_model.fit(
run_imgs=adhd_dataset.func[0], design_matrices=design_matrix
)
/home/remi/github/nilearn/nilearn_doc_build/.tox/doc/lib/python3.9/site-packages/nilearn/glm/first_level/first_level.py:585: UserWarning:
Mean values of 0 observed. The data have probably been centered.Scaling might not work as expected
Estimate the contrast.
print("Contrast seed_based_glm computed.")
z_map = first_level_model.compute_contrast(
contrasts["seed_based_glm"], output_type="z_score"
)
# Saving snapshots of the contrasts
filename = "dmn_z_map.png"
display = plotting.plot_stat_map(
z_map, threshold=3.0, title="Seed based GLM", cut_coords=pcc_coords
)
display.add_markers(
marker_coords=[pcc_coords], marker_color="g", marker_size=300
)
display.savefig(filename)
print(f"Save z-map in '{filename}'.")
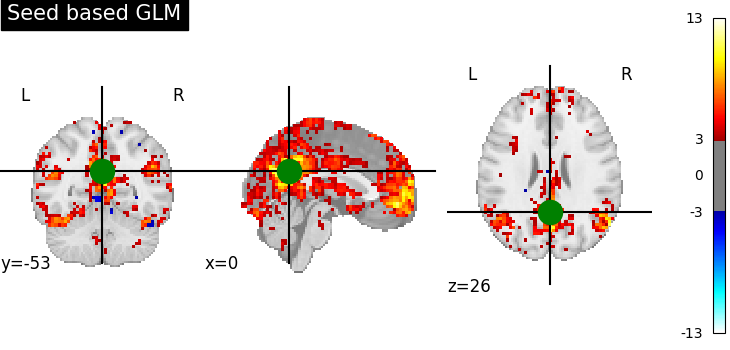
Contrast seed_based_glm computed.
Save z-map in 'dmn_z_map.png'.
Generating a report¶
It can be useful to quickly generate a portable, ready-to-view report with most of the pertinent information. This is easy to do if you have a fitted model and the list of contrasts, which we do here.
from nilearn.reporting import make_glm_report
report = make_glm_report(
first_level_model,
contrasts=contrasts,
title="ADHD DMN Report",
cluster_threshold=15,
min_distance=8.0,
plot_type="glass",
)
We have several ways to access the report:
# report # This report can be viewed in a notebook
# report.open_in_browser()
# or we can save as an html file
# from pathlib import Path
# output_dir = Path.cwd() / "results" / "plot_adhd_dmn"
# output_dir.mkdir(exist_ok=True, parents=True)
# report.save_as_html(output_dir / 'report.html')
Total running time of the script: (0 minutes 23.371 seconds)
Estimated memory usage: 1015 MB