Note
Go to the end to download the full example code. or to run this example in your browser via Binder
Setting a parameter by cross-validation¶
Here we set the number of features selected in an Anova-SVC approach to maximize the cross-validation score.
After separating 2 runs for validation, we vary that parameter and measure the cross-validation score. We also measure the prediction score on the left-out validation data. As we can see, the two scores vary by a significant amount: this is due to sampling noise in cross validation, and choosing the parameter k to maximize the cross-validation score, might not maximize the score on left-out data.
Thus using data to maximize a cross-validation score computed on that same data is likely to be too optimistic and lead to an overfit.
The proper approach is known as a “nested cross-validation”. It consists in doing cross-validation loops to set the model parameters inside the cross-validation loop used to judge the prediction performance: the parameters are set separately on each fold, never using the data used to measure performance.
For decoding tasks, in nilearn, this can be done using the
Decoder
object, which will automatically select
the best parameters of an estimator from a grid of parameter values.
One difficulty is that the Decoder object is a composite estimator: a pipeline of feature selection followed by Support Vector Machine. Tuning the SVM’s parameters is already done automatically inside the Decoder, but performing cross-validation for the feature selection must be done manually.
Load the Haxby dataset¶
from nilearn import datasets
from nilearn.plotting import show
# by default 2nd subject data will be fetched on which we run our analysis
haxby_dataset = datasets.fetch_haxby()
fmri_img = haxby_dataset.func[0]
mask_img = haxby_dataset.mask
# print basic information on the dataset
print(f"Mask nifti image (3D) is located at: {haxby_dataset.mask}")
print(f"Functional nifti image (4D) are located at: {haxby_dataset.func[0]}")
# Load the behavioral data
import pandas as pd
labels = pd.read_csv(haxby_dataset.session_target[0], sep=" ")
y = labels["labels"]
# Keep only data corresponding to shoes or bottles
from nilearn.image import index_img
condition_mask = y.isin(["shoe", "bottle"])
fmri_niimgs = index_img(fmri_img, condition_mask)
y = y[condition_mask]
run = labels["chunks"][condition_mask]
[get_dataset_dir] Dataset found in /home/runner/nilearn_data/haxby2001
Mask nifti image (3D) is located at: /home/runner/nilearn_data/haxby2001/mask.nii.gz
Functional nifti image (4D) are located at: /home/runner/nilearn_data/haxby2001/subj2/bold.nii.gz
ANOVA pipeline with Decoder
object¶
Nilearn Decoder object aims to provide smooth user experience by acting as a pipeline of several tasks: preprocessing with NiftiMasker, reducing dimension by selecting only relevant features with ANOVA – a classical univariate feature selection based on F-test, and then decoding with different types of estimators (in this example is Support Vector Machine with a linear kernel) on nested cross-validation.
from nilearn.decoding import Decoder
# We provide a grid of hyperparameter values to the Decoder's internal
# cross-validation. If no param_grid is provided, the Decoder will use a
# default grid with sensible values for the chosen estimator
param_grid = [
{
"penalty": ["l2"],
"dual": [True],
"C": [100, 1000],
},
{
"penalty": ["l1"],
"dual": [False],
"C": [100, 1000],
},
]
# Here screening_percentile is set to 2 percent, meaning around 800
# features will be selected with ANOVA.
decoder = Decoder(
estimator="svc",
cv=5,
mask=mask_img,
smoothing_fwhm=4,
standardize="zscore_sample",
screening_percentile=2,
param_grid=param_grid,
)
Fit the Decoder and predict the responses¶
As a complete pipeline by itself, decoder will perform cross-validation for the estimator, in this case Support Vector Machine. We can output the best parameters selected for each cross-validation fold. See https://scikit-learn.org/stable/modules/cross_validation.html for an excellent explanation of how cross-validation works.
# Fit the Decoder
decoder.fit(fmri_niimgs, y)
# Print the best parameters for each fold
for i, (best_c, best_penalty, best_dual, cv_score) in enumerate(
zip(
decoder.cv_params_["shoe"]["C"],
decoder.cv_params_["shoe"]["penalty"],
decoder.cv_params_["shoe"]["dual"],
decoder.cv_scores_["shoe"],
)
):
print(
f"Fold {i + 1} | Best SVM parameters: C={best_c}"
f", penalty={best_penalty}, dual={best_dual} with score: {cv_score}"
)
# Output the prediction with Decoder
y_pred = decoder.predict(fmri_niimgs)
Fold 1 | Best SVM parameters: C=1000, penalty=l1, dual=False with score: 0.9256198347107438
Fold 2 | Best SVM parameters: C=1000, penalty=l2, dual=True with score: 0.9177489177489176
Fold 3 | Best SVM parameters: C=100, penalty=l1, dual=False with score: 0.7965367965367965
Fold 4 | Best SVM parameters: C=100, penalty=l1, dual=False with score: 0.7792207792207793
Fold 5 | Best SVM parameters: C=1000, penalty=l2, dual=True with score: 0.735930735930736
Compute prediction scores with different values of screening percentile¶
import numpy as np
screening_percentile_range = [2, 4, 8, 16, 32, 64]
cv_scores = []
val_scores = []
for sp in screening_percentile_range:
decoder = Decoder(
estimator="svc",
mask=mask_img,
smoothing_fwhm=4,
cv=3,
standardize="zscore_sample",
screening_percentile=sp,
param_grid=param_grid,
)
decoder.fit(index_img(fmri_niimgs, run < 10), y[run < 10])
cv_scores.append(np.mean(decoder.cv_scores_["bottle"]))
print(f"Sreening Percentile: {sp:.3f}")
print(f"Mean CV score: {cv_scores[-1]:.4f}")
y_pred = decoder.predict(index_img(fmri_niimgs, run == 10))
val_scores.append(np.mean(y_pred == y[run == 10]))
print(f"Validation score: {val_scores[-1]:.4f}")
Sreening Percentile: 2.000
Mean CV score: 0.8456
Validation score: 0.3889
Sreening Percentile: 4.000
Mean CV score: 0.8493
Validation score: 0.3889
Sreening Percentile: 8.000
Mean CV score: 0.8548
Validation score: 0.7222
Sreening Percentile: 16.000
Mean CV score: 0.8767
Validation score: 0.2778
Sreening Percentile: 32.000
Mean CV score: 0.8715
Validation score: 0.3889
Sreening Percentile: 64.000
Mean CV score: 0.8974
Validation score: 0.4444
Nested cross-validation¶
We are going to tune the parameter ‘screening_percentile’ in the pipeline.
from sklearn.model_selection import KFold
cv = KFold(n_splits=3)
nested_cv_scores = []
for train, test in cv.split(run):
y_train = np.array(y)[train]
y_test = np.array(y)[test]
val_scores = []
for sp in screening_percentile_range:
decoder = Decoder(
estimator="svc",
mask=mask_img,
smoothing_fwhm=4,
cv=3,
standardize="zscore_sample",
screening_percentile=sp,
param_grid=param_grid,
)
decoder.fit(index_img(fmri_niimgs, train), y_train)
y_pred = decoder.predict(index_img(fmri_niimgs, test))
val_scores.append(np.mean(y_pred == y_test))
nested_cv_scores.append(np.max(val_scores))
print(f"Nested CV score: {np.mean(nested_cv_scores):.4f}")
/home/runner/work/nilearn/nilearn/.tox/doc/lib/python3.9/site-packages/sklearn/svm/_base.py:1237: ConvergenceWarning: Liblinear failed to converge, increase the number of iterations.
warnings.warn(
/home/runner/work/nilearn/nilearn/.tox/doc/lib/python3.9/site-packages/sklearn/svm/_base.py:1237: ConvergenceWarning: Liblinear failed to converge, increase the number of iterations.
warnings.warn(
/home/runner/work/nilearn/nilearn/.tox/doc/lib/python3.9/site-packages/sklearn/svm/_base.py:1237: ConvergenceWarning: Liblinear failed to converge, increase the number of iterations.
warnings.warn(
/home/runner/work/nilearn/nilearn/.tox/doc/lib/python3.9/site-packages/sklearn/svm/_base.py:1237: ConvergenceWarning: Liblinear failed to converge, increase the number of iterations.
warnings.warn(
/home/runner/work/nilearn/nilearn/.tox/doc/lib/python3.9/site-packages/sklearn/svm/_base.py:1237: ConvergenceWarning: Liblinear failed to converge, increase the number of iterations.
warnings.warn(
/home/runner/work/nilearn/nilearn/.tox/doc/lib/python3.9/site-packages/sklearn/svm/_base.py:1237: ConvergenceWarning: Liblinear failed to converge, increase the number of iterations.
warnings.warn(
/home/runner/work/nilearn/nilearn/.tox/doc/lib/python3.9/site-packages/sklearn/svm/_base.py:1237: ConvergenceWarning: Liblinear failed to converge, increase the number of iterations.
warnings.warn(
/home/runner/work/nilearn/nilearn/.tox/doc/lib/python3.9/site-packages/sklearn/svm/_base.py:1237: ConvergenceWarning: Liblinear failed to converge, increase the number of iterations.
warnings.warn(
/home/runner/work/nilearn/nilearn/.tox/doc/lib/python3.9/site-packages/sklearn/svm/_base.py:1237: ConvergenceWarning: Liblinear failed to converge, increase the number of iterations.
warnings.warn(
/home/runner/work/nilearn/nilearn/.tox/doc/lib/python3.9/site-packages/sklearn/svm/_base.py:1237: ConvergenceWarning: Liblinear failed to converge, increase the number of iterations.
warnings.warn(
/home/runner/work/nilearn/nilearn/.tox/doc/lib/python3.9/site-packages/sklearn/svm/_base.py:1237: ConvergenceWarning: Liblinear failed to converge, increase the number of iterations.
warnings.warn(
/home/runner/work/nilearn/nilearn/.tox/doc/lib/python3.9/site-packages/sklearn/svm/_base.py:1237: ConvergenceWarning: Liblinear failed to converge, increase the number of iterations.
warnings.warn(
/home/runner/work/nilearn/nilearn/.tox/doc/lib/python3.9/site-packages/sklearn/svm/_base.py:1237: ConvergenceWarning: Liblinear failed to converge, increase the number of iterations.
warnings.warn(
/home/runner/work/nilearn/nilearn/.tox/doc/lib/python3.9/site-packages/sklearn/svm/_base.py:1237: ConvergenceWarning: Liblinear failed to converge, increase the number of iterations.
warnings.warn(
Nested CV score: 0.6898
Plot the prediction scores using matplotlib¶
from matplotlib import pyplot as plt
plt.figure(figsize=(6, 4))
plt.plot(cv_scores, label="Cross validation scores")
plt.plot(val_scores, label="Left-out validation data scores")
plt.xticks(
np.arange(len(screening_percentile_range)), screening_percentile_range
)
plt.axis("tight")
plt.xlabel("ANOVA screening percentile")
plt.axhline(
np.mean(nested_cv_scores), label="Nested cross-validation", color="r"
)
plt.legend(loc="best", frameon=False)
show()
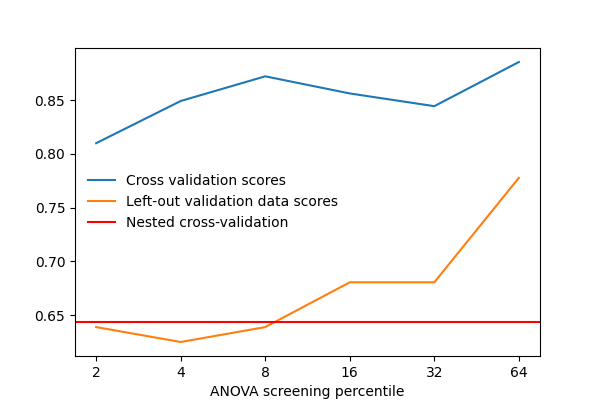
Total running time of the script: (2 minutes 24.156 seconds)
Estimated memory usage: 1009 MB