Note
Go to the end to download the full example code or to run this example in your browser via Binder
Encoding models for visual stimuli from Miyawaki et al. 2008#
- This example partly reproduces the encoding model presented in
Visual image reconstruction from human brain activity using a combination of multiscale local image decoders, Miyawaki, Y., Uchida, H., Yamashita, O., Sato, M. A., Morito, Y., Tanabe, H. C., … & Kamitani, Y. (2008). Neuron, 60(5), 915-929.
Encoding models try to predict neuronal activity using information from presented stimuli, like an image or sound. Where decoding goes from brain data to real-world stimulus, encoding goes the other direction.
We demonstrate how to build such an encoding model in nilearn, predicting fMRI data from visual stimuli, using the dataset from Miyawaki et al., 2008.
Participants were shown images, which consisted of random 10x10 binary (either black or white) pixels, and the corresponding fMRI activity was recorded. We will try to predict the activity in each voxel from the binary pixel-values of the presented images. Then we extract the receptive fields for a set of voxels to see which pixel location a voxel is most sensitive to.
See also Reconstruction of visual stimuli from Miyawaki et al. 2008 for a decoding approach for the same dataset.
Note
If you are using Nilearn with a version older than 0.9.0
, then you should either upgrade your version or import maskers from the input_data
module instead of the maskers
module.
That is, you should manually replace in the following example all occurrences of:
from nilearn.maskers import NiftiMasker
with:
from nilearn.input_data import NiftiMasker
Loading the data#
Now we can load the data set:
from nilearn.datasets import fetch_miyawaki2008
dataset = fetch_miyawaki2008()
Dataset created in /home/remi/nilearn_data/miyawaki2008
Downloading data from https://www.nitrc.org/frs/download.php/8486/miyawaki2008.tgz ...
Downloaded 1441792 of 161069109 bytes (0.9%, 1.8min remaining)
Downloaded 3432448 of 161069109 bytes (2.1%, 1.5min remaining)
Downloaded 5570560 of 161069109 bytes (3.5%, 1.4min remaining)
Downloaded 7266304 of 161069109 bytes (4.5%, 1.5min remaining)
Downloaded 8658944 of 161069109 bytes (5.4%, 1.5min remaining)
Downloaded 10043392 of 161069109 bytes (6.2%, 1.5min remaining)
Downloaded 11411456 of 161069109 bytes (7.1%, 1.6min remaining)
Downloaded 12845056 of 161069109 bytes (8.0%, 1.6min remaining)
Downloaded 14295040 of 161069109 bytes (8.9%, 1.6min remaining)
Downloaded 15818752 of 161069109 bytes (9.8%, 1.6min remaining)
Downloaded 17416192 of 161069109 bytes (10.8%, 1.5min remaining)
Downloaded 19087360 of 161069109 bytes (11.9%, 1.5min remaining)
Downloaded 20652032 of 161069109 bytes (12.8%, 1.5min remaining)
Downloaded 21905408 of 161069109 bytes (13.6%, 1.5min remaining)
Downloaded 23166976 of 161069109 bytes (14.4%, 1.5min remaining)
Downloaded 24444928 of 161069109 bytes (15.2%, 1.5min remaining)
Downloaded 25403392 of 161069109 bytes (15.8%, 1.5min remaining)
Downloaded 26312704 of 161069109 bytes (16.3%, 1.6min remaining)
Downloaded 27238400 of 161069109 bytes (16.9%, 1.6min remaining)
Downloaded 28196864 of 161069109 bytes (17.5%, 1.6min remaining)
Downloaded 29089792 of 161069109 bytes (18.1%, 1.6min remaining)
Downloaded 29851648 of 161069109 bytes (18.5%, 1.6min remaining)
Downloaded 30711808 of 161069109 bytes (19.1%, 1.7min remaining)
Downloaded 31293440 of 161069109 bytes (19.4%, 1.7min remaining)
Downloaded 31891456 of 161069109 bytes (19.8%, 1.7min remaining)
Downloaded 32382976 of 161069109 bytes (20.1%, 1.8min remaining)
Downloaded 32923648 of 161069109 bytes (20.4%, 1.8min remaining)
Downloaded 33529856 of 161069109 bytes (20.8%, 1.8min remaining)
Downloaded 34127872 of 161069109 bytes (21.2%, 1.8min remaining)
Downloaded 34783232 of 161069109 bytes (21.6%, 1.9min remaining)
Downloaded 35504128 of 161069109 bytes (22.0%, 1.9min remaining)
Downloaded 36290560 of 161069109 bytes (22.5%, 1.9min remaining)
Downloaded 37150720 of 161069109 bytes (23.1%, 1.9min remaining)
Downloaded 38297600 of 161069109 bytes (23.8%, 1.9min remaining)
Downloaded 39501824 of 161069109 bytes (24.5%, 1.8min remaining)
Downloaded 40763392 of 161069109 bytes (25.3%, 1.8min remaining)
Downloaded 42180608 of 161069109 bytes (26.2%, 1.8min remaining)
Downloaded 43147264 of 161069109 bytes (26.8%, 1.8min remaining)
Downloaded 44032000 of 161069109 bytes (27.3%, 1.8min remaining)
Downloaded 44933120 of 161069109 bytes (27.9%, 1.8min remaining)
Downloaded 45817856 of 161069109 bytes (28.4%, 1.7min remaining)
Downloaded 46759936 of 161069109 bytes (29.0%, 1.7min remaining)
Downloaded 47759360 of 161069109 bytes (29.7%, 1.7min remaining)
Downloaded 48848896 of 161069109 bytes (30.3%, 1.7min remaining)
Downloaded 49995776 of 161069109 bytes (31.0%, 1.7min remaining)
Downloaded 51232768 of 161069109 bytes (31.8%, 1.7min remaining)
Downloaded 52830208 of 161069109 bytes (32.8%, 1.6min remaining)
Downloaded 54763520 of 161069109 bytes (34.0%, 1.6min remaining)
Downloaded 56197120 of 161069109 bytes (34.9%, 1.6min remaining)
Downloaded 57499648 of 161069109 bytes (35.7%, 1.5min remaining)
Downloaded 58695680 of 161069109 bytes (36.4%, 1.5min remaining)
Downloaded 59588608 of 161069109 bytes (37.0%, 1.5min remaining)
Downloaded 60276736 of 161069109 bytes (37.4%, 1.5min remaining)
Downloaded 61030400 of 161069109 bytes (37.9%, 1.5min remaining)
Downloaded 61816832 of 161069109 bytes (38.4%, 1.5min remaining)
Downloaded 62660608 of 161069109 bytes (38.9%, 1.5min remaining)
Downloaded 63569920 of 161069109 bytes (39.5%, 1.5min remaining)
Downloaded 64544768 of 161069109 bytes (40.1%, 1.5min remaining)
Downloaded 65413120 of 161069109 bytes (40.6%, 1.5min remaining)
Downloaded 66314240 of 161069109 bytes (41.2%, 1.5min remaining)
Downloaded 67297280 of 161069109 bytes (41.8%, 1.4min remaining)
Downloaded 68313088 of 161069109 bytes (42.4%, 1.4min remaining)
Downloaded 69369856 of 161069109 bytes (43.1%, 1.4min remaining)
Downloaded 70418432 of 161069109 bytes (43.7%, 1.4min remaining)
Downloaded 71499776 of 161069109 bytes (44.4%, 1.4min remaining)
Downloaded 72704000 of 161069109 bytes (45.1%, 1.4min remaining)
Downloaded 74113024 of 161069109 bytes (46.0%, 1.3min remaining)
Downloaded 75882496 of 161069109 bytes (47.1%, 1.3min remaining)
Downloaded 77086720 of 161069109 bytes (47.9%, 1.3min remaining)
Downloaded 78209024 of 161069109 bytes (48.6%, 1.3min remaining)
Downloaded 79486976 of 161069109 bytes (49.3%, 1.2min remaining)
Downloaded 80576512 of 161069109 bytes (50.0%, 1.2min remaining)
Downloaded 81649664 of 161069109 bytes (50.7%, 1.2min remaining)
Downloaded 82493440 of 161069109 bytes (51.2%, 1.2min remaining)
Downloaded 83451904 of 161069109 bytes (51.8%, 1.2min remaining)
Downloaded 84410368 of 161069109 bytes (52.4%, 1.2min remaining)
Downloaded 85434368 of 161069109 bytes (53.0%, 1.2min remaining)
Downloaded 86499328 of 161069109 bytes (53.7%, 1.1min remaining)
Downloaded 87678976 of 161069109 bytes (54.4%, 1.1min remaining)
Downloaded 88907776 of 161069109 bytes (55.2%, 1.1min remaining)
Downloaded 90324992 of 161069109 bytes (56.1%, 1.1min remaining)
Downloaded 92102656 of 161069109 bytes (57.2%, 1.0min remaining)
Downloaded 93945856 of 161069109 bytes (58.3%, 1.0min remaining)
Downloaded 95248384 of 161069109 bytes (59.1%, 59.0s remaining)
Downloaded 96649216 of 161069109 bytes (60.0%, 57.7s remaining)
Downloaded 97894400 of 161069109 bytes (60.8%, 56.5s remaining)
Downloaded 99106816 of 161069109 bytes (61.5%, 55.3s remaining)
Downloaded 100360192 of 161069109 bytes (62.3%, 54.1s remaining)
Downloaded 101629952 of 161069109 bytes (63.1%, 52.9s remaining)
Downloaded 102932480 of 161069109 bytes (63.9%, 51.7s remaining)
Downloaded 104292352 of 161069109 bytes (64.8%, 50.4s remaining)
Downloaded 105717760 of 161069109 bytes (65.6%, 49.0s remaining)
Downloaded 107331584 of 161069109 bytes (66.6%, 47.3s remaining)
Downloaded 109232128 of 161069109 bytes (67.8%, 45.3s remaining)
Downloaded 111214592 of 161069109 bytes (69.0%, 43.3s remaining)
Downloaded 112959488 of 161069109 bytes (70.1%, 41.5s remaining)
Downloaded 114532352 of 161069109 bytes (71.1%, 40.1s remaining)
Downloaded 116162560 of 161069109 bytes (72.1%, 38.5s remaining)
Downloaded 117891072 of 161069109 bytes (73.2%, 36.8s remaining)
Downloaded 119480320 of 161069109 bytes (74.2%, 35.4s remaining)
Downloaded 120946688 of 161069109 bytes (75.1%, 34.1s remaining)
Downloaded 122183680 of 161069109 bytes (75.9%, 33.0s remaining)
Downloaded 123387904 of 161069109 bytes (76.6%, 32.0s remaining)
Downloaded 124649472 of 161069109 bytes (77.4%, 30.9s remaining)
Downloaded 125919232 of 161069109 bytes (78.2%, 29.8s remaining)
Downloaded 127221760 of 161069109 bytes (79.0%, 28.7s remaining)
Downloaded 128622592 of 161069109 bytes (79.9%, 27.4s remaining)
Downloaded 130048000 of 161069109 bytes (80.7%, 26.2s remaining)
Downloaded 131620864 of 161069109 bytes (81.7%, 24.8s remaining)
Downloaded 133193728 of 161069109 bytes (82.7%, 23.4s remaining)
Downloaded 134914048 of 161069109 bytes (83.8%, 21.9s remaining)
Downloaded 136658944 of 161069109 bytes (84.8%, 20.3s remaining)
Downloaded 138100736 of 161069109 bytes (85.7%, 19.1s remaining)
Downloaded 139583488 of 161069109 bytes (86.7%, 17.8s remaining)
Downloaded 141156352 of 161069109 bytes (87.6%, 16.5s remaining)
Downloaded 142704640 of 161069109 bytes (88.6%, 15.2s remaining)
Downloaded 144252928 of 161069109 bytes (89.6%, 13.9s remaining)
Downloaded 145850368 of 161069109 bytes (90.6%, 12.5s remaining)
Downloaded 147537920 of 161069109 bytes (91.6%, 11.1s remaining)
Downloaded 148897792 of 161069109 bytes (92.4%, 10.0s remaining)
Downloaded 150462464 of 161069109 bytes (93.4%, 8.7s remaining)
Downloaded 152141824 of 161069109 bytes (94.5%, 7.3s remaining)
Downloaded 153862144 of 161069109 bytes (95.5%, 5.8s remaining)
Downloaded 155238400 of 161069109 bytes (96.4%, 4.7s remaining)
Downloaded 156762112 of 161069109 bytes (97.3%, 3.5s remaining)
Downloaded 158425088 of 161069109 bytes (98.4%, 2.1s remaining)
Downloaded 160104448 of 161069109 bytes (99.4%, 0.8s remaining) ...done. (130 seconds, 2 min)
Extracting data from /home/remi/nilearn_data/miyawaki2008/18b67c55cebe5e71427c5ffdcfafd948/miyawaki2008.tgz..... done.
We only use the training data of this study, where random binary images were shown.
# training data starts after the first 12 files
fmri_random_runs_filenames = dataset.func[12:]
stimuli_random_runs_filenames = dataset.label[12:]
We can use nilearn.maskers.MultiNiftiMasker
to load the fMRI data, clean and mask it.
import numpy as np
from nilearn.maskers import MultiNiftiMasker
masker = MultiNiftiMasker(
mask_img=dataset.mask, detrend=True, standardize="zscore_sample"
)
masker.fit()
fmri_data = masker.transform(fmri_random_runs_filenames)
# shape of the binary (i.e. black and wihte values) image in pixels
stimulus_shape = (10, 10)
# We load the visual stimuli from csv files
stimuli = []
for stimulus_run in stimuli_random_runs_filenames:
stimuli.append(
np.reshape(
np.loadtxt(stimulus_run, dtype=int, delimiter=","),
(-1,) + stimulus_shape,
order="F",
)
)
Let’s take a look at some of these binary images:
import pylab as plt
plt.figure(figsize=(8, 4))
plt.subplot(1, 2, 1)
plt.imshow(stimuli[0][124], interpolation="nearest", cmap="gray")
plt.axis("off")
plt.title(f"Run {1}, Stimulus {125}")
plt.subplot(1, 2, 2)
plt.imshow(stimuli[2][101], interpolation="nearest", cmap="gray")
plt.axis("off")
plt.title(f"Run {3}, Stimulus {102}")
plt.subplots_adjust(wspace=0.5)
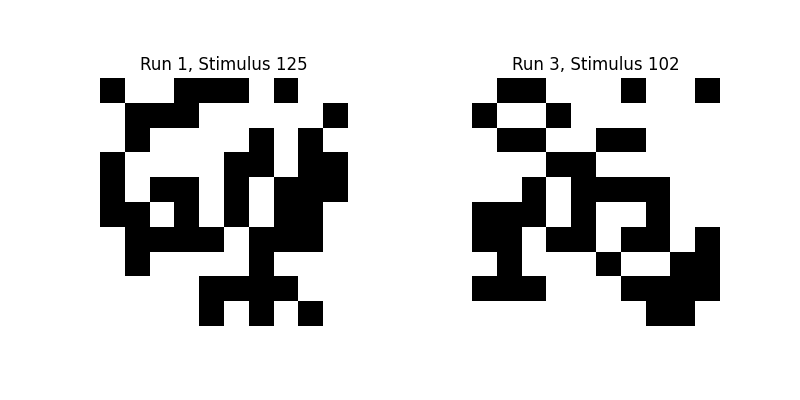
We now stack the fmri and stimulus data and remove an offset in the beginning/end.
fmri_data is a matrix of samples x voxels
print(fmri_data.shape)
(2860, 5438)
We flatten the last two dimensions of stimuli so it is a matrix of samples x pixels.
# Flatten the stimuli
stimuli = np.reshape(stimuli, (-1, stimulus_shape[0] * stimulus_shape[1]))
print(stimuli.shape)
(2860, 100)
Building the encoding models#
We can now proceed to build a simple voxel-wise encoding model using Ridge regression. For each voxel we fit an independent regression model, using the pixel-values of the visual stimuli to predict the neuronal activity in this voxel.
Using 10-fold cross-validation, we partition the data into 10 ‘folds’. We hold out each fold of the data for testing, then fit a ridge regression to the remaining 9/10 of the data, using stimuli as predictors and fmri_data as targets, and create predictions for the held-out 10th.
from sklearn.linear_model import Ridge
from sklearn.metrics import r2_score
from sklearn.model_selection import KFold
estimator = Ridge(alpha=100.0)
cv = KFold(n_splits=10)
scores = []
for train, test in cv.split(X=stimuli):
# we train the Ridge estimator on the training set
# and predict the fMRI activity for the test set
predictions = estimator.fit(
stimuli.reshape(-1, 100)[train], fmri_data[train]
).predict(stimuli.reshape(-1, 100)[test])
# we compute how much variance our encoding model explains in each voxel
scores.append(
r2_score(fmri_data[test], predictions, multioutput="raw_values")
)
Mapping the encoding scores on the brain#
To plot the scores onto the brain, we create a Nifti1Image containing the scores and then threshold it:
from nilearn.image import threshold_img
cut_score = np.mean(scores, axis=0)
cut_score[cut_score < 0] = 0
# bring the scores into the shape of the background brain
score_map_img = masker.inverse_transform(cut_score)
thresholded_score_map_img = threshold_img(
score_map_img, threshold=1e-6, copy=False
)
Plotting the statistical map on a background brain, we mark four voxels which we will inspect more closely later on.
from nilearn.image import coord_transform
from nilearn.plotting import plot_stat_map
def index_to_xy_coord(x, y, z=10):
"""Transform data index to coordinates of the background + offset."""
coords = coord_transform(x, y, z, affine=thresholded_score_map_img.affine)
return np.array(coords)[np.newaxis, :] + np.array([0, 1, 0])
xy_indices_of_special_voxels = [(30, 10), (32, 10), (31, 9), (31, 10)]
display = plot_stat_map(
thresholded_score_map_img,
bg_img=dataset.background,
cut_coords=[-8],
display_mode="z",
aspect=1.25,
title="Explained variance per voxel",
)
# creating a marker for each voxel and adding it to the statistical map
for i, (x, y) in enumerate(xy_indices_of_special_voxels):
display.add_markers(
index_to_xy_coord(x, y),
marker_color="none",
edgecolor=["b", "r", "magenta", "g"][i],
marker_size=140,
marker="s",
facecolor="none",
lw=4.5,
)
# re-set figure size after construction so colorbar gets rescaled too
fig = plt.gcf()
fig.set_size_inches(12, 12)
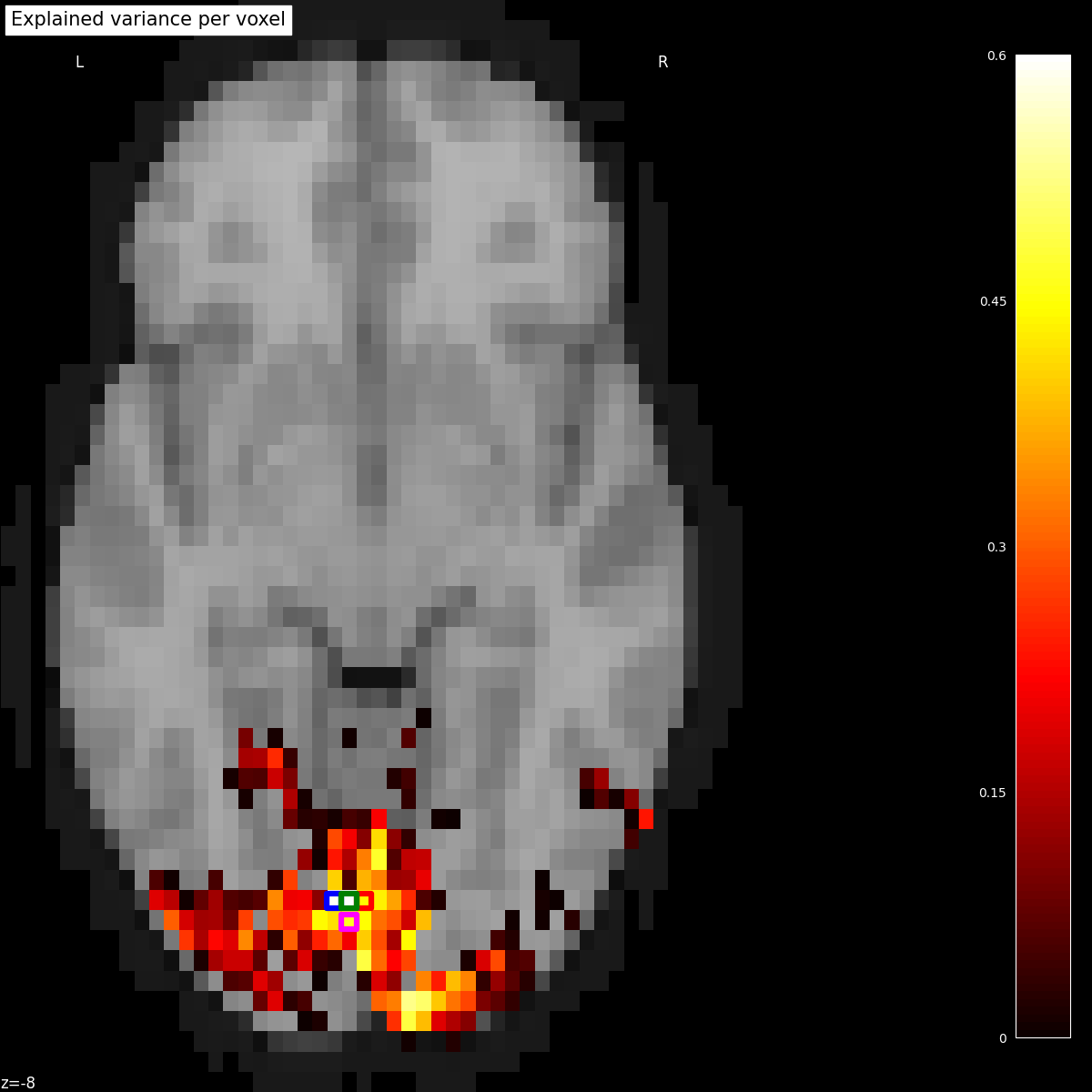
Estimating receptive fields#
Now we take a closer look at the receptive fields of the four marked voxels. A voxel’s receptive field is the region of a stimulus (like an image) where the presence of an object, like a white instead of a black pixel, results in a change in activity in the voxel. In our case the receptive field is just the vector of 100 regression coefficients (one for each pixel) reshaped into the 10x10 form of the original images. Some voxels are receptive to only very few pixels, so we use Lasso regression to estimate a sparse set of regression coefficients.
from sklearn.linear_model import LassoLarsCV
from sklearn.pipeline import make_pipeline
from sklearn.preprocessing import StandardScaler
# automatically estimate the sparsity by cross-validation
lasso = make_pipeline(StandardScaler(), LassoLarsCV(max_iter=10))
# Mark the same pixel in each receptive field
marked_pixel = (4, 2)
from matplotlib import gridspec
from matplotlib.patches import Rectangle
fig = plt.figure(figsize=(12, 8))
fig.suptitle("Receptive fields of the marked voxels", fontsize=25)
# GridSpec allows us to do subplots with more control of the spacing
gs1 = gridspec.GridSpec(2, 3)
# we fit the Lasso for each of the three voxels of the upper row
for i, index in enumerate([1780, 1951, 2131]):
ax = plt.subplot(gs1[0, i])
lasso.fit(stimuli, fmri_data[:, index])
# we reshape the coefficients into the form of the original images
rf = lasso.named_steps["lassolarscv"].coef_.reshape((10, 10))
# add a black background
ax.imshow(np.zeros_like(rf), vmin=0.0, vmax=1.0, cmap="gray")
ax_im = ax.imshow(
np.ma.masked_less(rf, 0.1),
interpolation="nearest",
cmap=["Blues", "Greens", "Reds"][i],
vmin=0.0,
vmax=0.75,
)
# add the marked pixel
ax.add_patch(
Rectangle(
(marked_pixel[1] - 0.5, marked_pixel[0] - 0.5),
1,
1,
facecolor="none",
edgecolor="r",
lw=4,
)
)
plt.axis("off")
plt.colorbar(ax_im, ax=ax)
# and then for the voxel at the bottom
gs1.update(left=0.0, right=1.0, wspace=0.1)
ax = plt.subplot(gs1[1, 1])
lasso.fit(stimuli, fmri_data[:, 1935])
# we reshape the coefficients into the form of the original images
rf = lasso.named_steps["lassolarscv"].coef_.reshape((10, 10))
ax.imshow(np.zeros_like(rf), vmin=0.0, vmax=1.0, cmap="gray")
ax_im = ax.imshow(
np.ma.masked_less(rf, 0.1),
interpolation="nearest",
cmap="RdPu",
vmin=0.0,
vmax=0.75,
)
# add the marked pixel
ax.add_patch(
Rectangle(
(marked_pixel[1] - 0.5, marked_pixel[0] - 0.5),
1,
1,
facecolor="none",
edgecolor="r",
lw=4,
)
)
plt.axis("off")
plt.colorbar(ax_im, ax=ax)
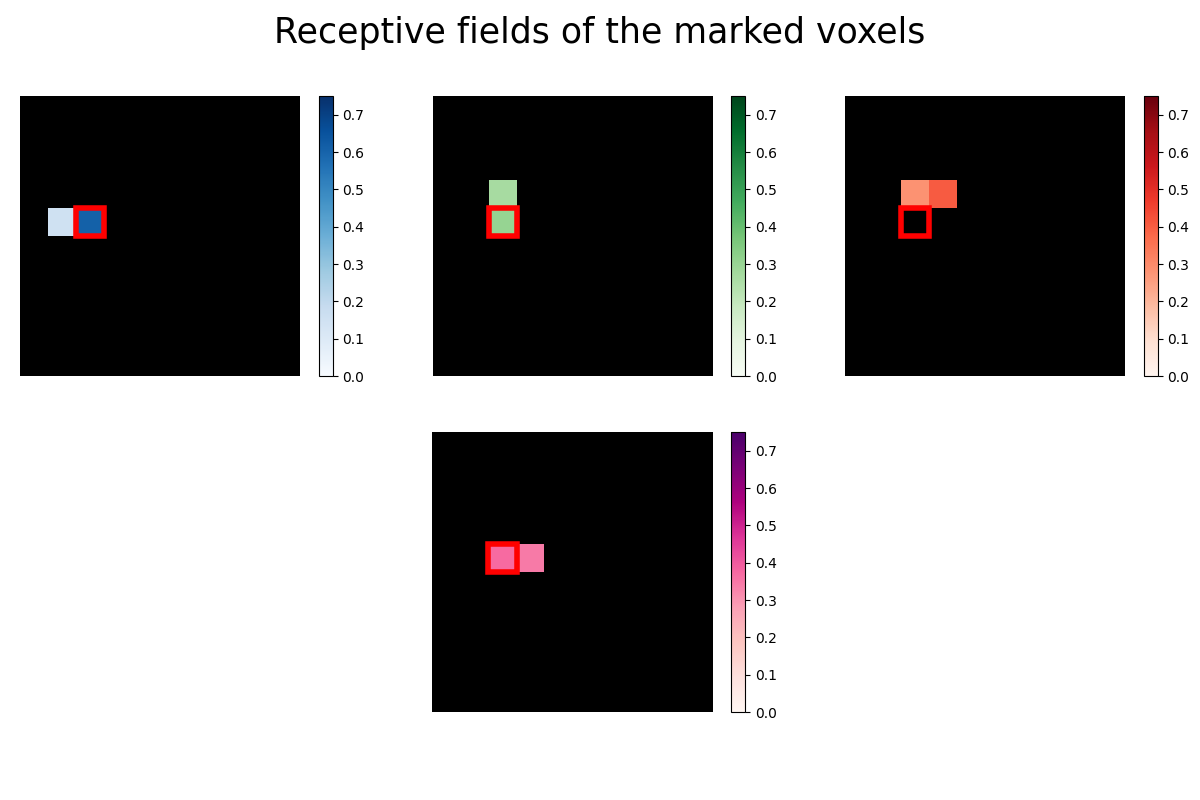
<matplotlib.colorbar.Colorbar object at 0x7fd1e3426ed0>
The receptive fields of the four voxels are not only close to each other, the relative location of the pixel each voxel is most sensitive to roughly maps to the relative location of the voxels to each other. We can see a relationship between some voxel’s receptive field and its location in the brain.
Total running time of the script: (2 minutes 37.655 seconds)
Estimated memory usage: 416 MB