Note
Click here to download the full example code or to run this example in your browser via Binder
9.4.2. Extracting signals from a brain parcellation¶
Here we show how to extract signals from a brain parcellation and compute a correlation matrix.
We also show the importance of defining good confounds signals: the first correlation matrix is computed after regressing out simple confounds signals: movement regressors, white matter and CSF signals, … The second one is without any confounds: all regions are connected to each other.
One reference that discusses the importance of confounds is Varoquaux and Craddock, Learning and comparing functional connectomes across subjects, NeuroImage 2013.
This is just a code example, see the corresponding section in the documentation for more.
Note
This example needs SciPy >= 1.0.0 for the reordering of the matrix.
9.4.2.1. Retrieve the atlas and the data¶
from nilearn import datasets
dataset = datasets.fetch_atlas_harvard_oxford('cort-maxprob-thr25-2mm')
atlas_filename = dataset.maps
labels = dataset.labels
print('Atlas ROIs are located in nifti image (4D) at: %s' %
atlas_filename) # 4D data
# One subject of brain development fmri data
data = datasets.fetch_development_fmri(n_subjects=1)
fmri_filenames = data.func[0]
Out:
Atlas ROIs are located in nifti image (4D) at: <class 'nibabel.nifti1.Nifti1Image'>
data shape (91, 109, 91)
affine:
[[ 2. 0. 0. -90.]
[ 0. 2. 0. -126.]
[ 0. 0. 2. -72.]
[ 0. 0. 0. 1.]]
metadata:
<class 'nibabel.nifti1.Nifti1Header'> object, endian='<'
sizeof_hdr : 348
data_type : b''
db_name : b''
extents : 0
session_error : 0
regular : b''
dim_info : 0
dim : [ 3 91 109 91 1 1 1 1]
intent_p1 : 0.0
intent_p2 : 0.0
intent_p3 : 0.0
intent_code : none
datatype : uint8
bitpix : 8
slice_start : 0
pixdim : [1. 2. 2. 2. 1. 1. 1. 1.]
vox_offset : 0.0
scl_slope : nan
scl_inter : nan
slice_end : 0
slice_code : unknown
xyzt_units : 0
cal_max : 0.0
cal_min : 0.0
slice_duration : 0.0
toffset : 0.0
glmax : 0
glmin : 0
descrip : b''
aux_file : b''
qform_code : unknown
sform_code : aligned
quatern_b : 0.0
quatern_c : 0.0
quatern_d : 0.0
qoffset_x : -90.0
qoffset_y : -126.0
qoffset_z : -72.0
srow_x : [ 2. 0. 0. -90.]
srow_y : [ 0. 2. 0. -126.]
srow_z : [ 0. 0. 2. -72.]
intent_name : b''
magic : b'n+1'
9.4.2.2. Extract signals on a parcellation defined by labels¶
Using the NiftiLabelsMasker
from nilearn.input_data import NiftiLabelsMasker
masker = NiftiLabelsMasker(labels_img=atlas_filename, standardize=True,
memory='nilearn_cache', verbose=5)
# Here we go from nifti files to the signal time series in a numpy
# array. Note how we give confounds to be regressed out during signal
# extraction
time_series = masker.fit_transform(fmri_filenames, confounds=data.confounds)
Out:
[NiftiLabelsMasker.fit_transform] loading data from Nifti1Image(
shape=(91, 109, 91),
affine=array([[ 2., 0., 0., -90.],
[ 0., 2., 0., -126.],
[ 0., 0., 2., -72.],
[ 0., 0., 0., 1.]])
)
Resampling labels
________________________________________________________________________________
[Memory] Calling nilearn.input_data.base_masker.filter_and_extract...
filter_and_extract('/home/nicolas/nilearn_data/development_fmri/development_fmri/sub-pixar123_task-pixar_space-MNI152NLin2009cAsym_desc-preproc_bold.nii.gz',
<nilearn.input_data.nifti_labels_masker._ExtractionFunctor object at 0x7fbefd3b80d0>,
{ 'background_label': 0,
'detrend': False,
'dtype': None,
'high_pass': None,
'high_variance_confounds': False,
'labels': None,
'labels_img': <nibabel.nifti1.Nifti1Image object at 0x7fbef948dee0>,
'low_pass': None,
'mask_img': None,
'reports': True,
'smoothing_fwhm': None,
'standardize': True,
'standardize_confounds': True,
'strategy': 'mean',
't_r': None,
'target_affine': None,
'target_shape': None}, confounds=[ '/home/nicolas/nilearn_data/development_fmri/development_fmri/sub-pixar123_task-pixar_desc-reducedConfounds_regressors.tsv'], sample_mask=None, dtype=None, memory=Memory(location=nilearn_cache/joblib), memory_level=1, verbose=5)
[NiftiLabelsMasker.transform_single_imgs] Loading data from /home/nicolas/nilearn_data/development_fmri/development_fmri/sub-pixar123_task-pixar_space-MNI152NLin2009cAsym_desc-preproc_bold.nii.gz
[NiftiLabelsMasker.transform_single_imgs] Extracting region signals
[NiftiLabelsMasker.transform_single_imgs] Cleaning extracted signals
_______________________________________________filter_and_extract - 0.7s, 0.0min
9.4.2.3. Compute and display a correlation matrix¶
from nilearn.connectome import ConnectivityMeasure
correlation_measure = ConnectivityMeasure(kind='correlation')
correlation_matrix = correlation_measure.fit_transform([time_series])[0]
# Plot the correlation matrix
import numpy as np
from nilearn import plotting
# Make a large figure
# Mask the main diagonal for visualization:
np.fill_diagonal(correlation_matrix, 0)
# The labels we have start with the background (0), hence we skip the
# first label
# matrices are ordered for block-like representation
plotting.plot_matrix(correlation_matrix, figure=(10, 8), labels=labels[1:],
vmax=0.8, vmin=-0.8, reorder=True)
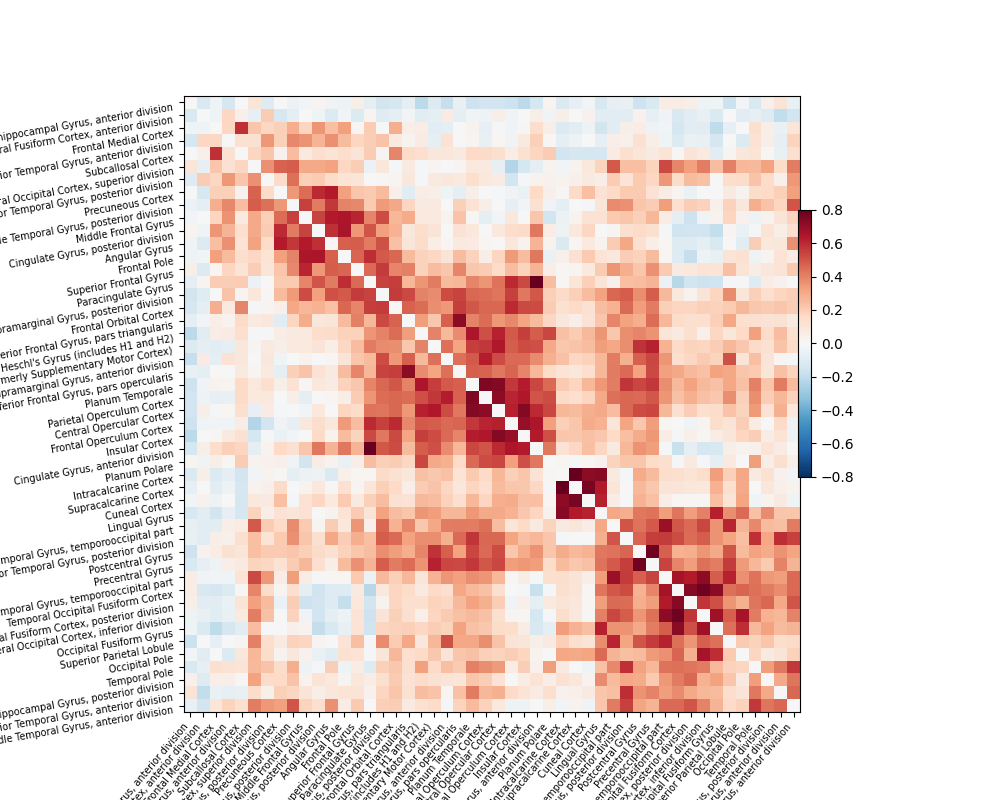
Out:
<matplotlib.image.AxesImage object at 0x7fbefe9c9dc0>
9.4.2.4. Same thing without confounds, to stress the importance of confounds¶
time_series = masker.fit_transform(fmri_filenames)
# Note how we did not specify confounds above. This is bad!
correlation_matrix = correlation_measure.fit_transform([time_series])[0]
# Mask the main diagonal for visualization:
np.fill_diagonal(correlation_matrix, 0)
plotting.plot_matrix(correlation_matrix, figure=(10, 8), labels=labels[1:],
vmax=0.8, vmin=-0.8, title='No confounds', reorder=True)
plotting.show()
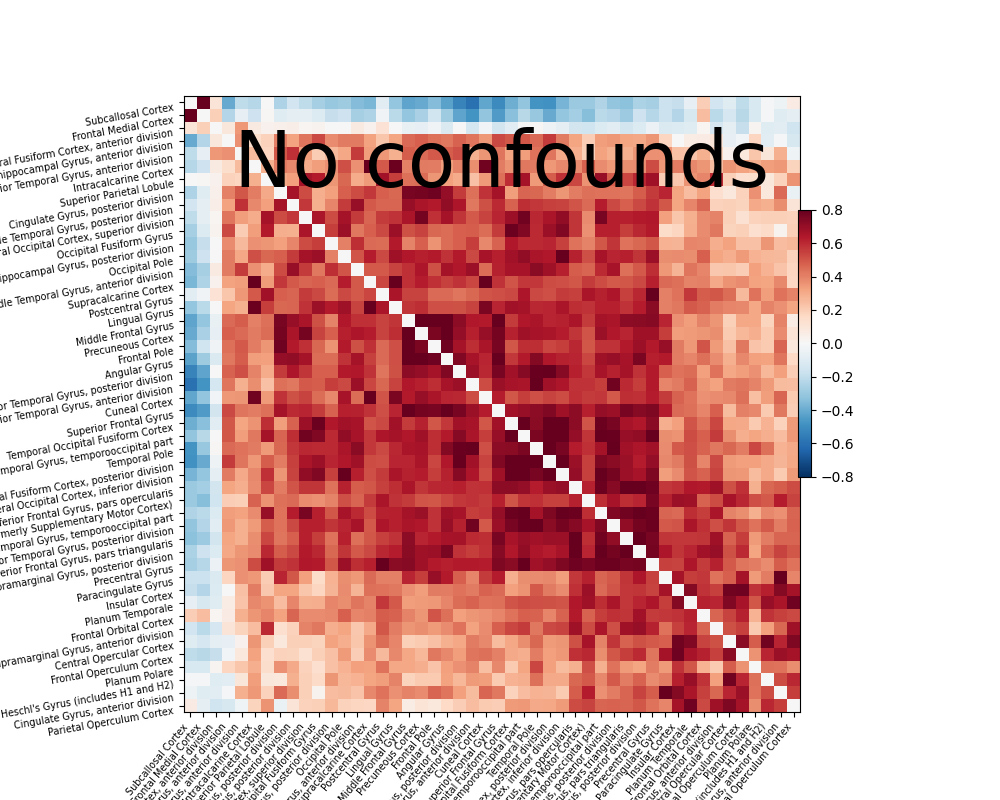
Out:
[NiftiLabelsMasker.fit_transform] loading data from Nifti1Image(
shape=(91, 109, 91),
affine=array([[ 2., 0., 0., -90.],
[ 0., 2., 0., -126.],
[ 0., 0., 2., -72.],
[ 0., 0., 0., 1.]])
)
________________________________________________________________________________
[Memory] Calling nilearn.input_data.base_masker.filter_and_extract...
filter_and_extract('/home/nicolas/nilearn_data/development_fmri/development_fmri/sub-pixar123_task-pixar_space-MNI152NLin2009cAsym_desc-preproc_bold.nii.gz',
<nilearn.input_data.nifti_labels_masker._ExtractionFunctor object at 0x7fbef4d305e0>,
{ 'background_label': 0,
'detrend': False,
'dtype': None,
'high_pass': None,
'high_variance_confounds': False,
'labels': None,
'labels_img': <nibabel.nifti1.Nifti1Image object at 0x7fbef948dee0>,
'low_pass': None,
'mask_img': None,
'reports': True,
'smoothing_fwhm': None,
'standardize': True,
'standardize_confounds': True,
'strategy': 'mean',
't_r': None,
'target_affine': None,
'target_shape': None}, confounds=None, sample_mask=None, dtype=None, memory=Memory(location=nilearn_cache/joblib), memory_level=1, verbose=5)
[NiftiLabelsMasker.transform_single_imgs] Loading data from /home/nicolas/nilearn_data/development_fmri/development_fmri/sub-pixar123_task-pixar_space-MNI152NLin2009cAsym_desc-preproc_bold.nii.gz
[NiftiLabelsMasker.transform_single_imgs] Extracting region signals
[NiftiLabelsMasker.transform_single_imgs] Cleaning extracted signals
_______________________________________________filter_and_extract - 0.7s, 0.0min
Total running time of the script: ( 0 minutes 2.727 seconds)